Datasets from CVPR 2023 publications are now browsable through FiftyOne Teams at the Voxel51 booth and at cvpr.fiftyone.ai
The annual IEEE/CVF Conference on Computer Vision and Pattern Recognition (CVPR) is here! Known for its prestigious nature and exceptional creativity, researchers from all over the world share their published findings while continuing to push the boundaries of computer vision, machine learning and AI. Recently, we published an interesting blog post analyzing the statistics behind this year’s papers. From there, we learned that out of 9,155 submissions, 2,359 papers were accepted to this year’s conference. After some investigating, we found that 59 of the 2,359 accepted papers were associated with a new dataset. That’s 2.5% — impressive, right?! As a team who has built multiple datasets of our own over the last few years, we know full well the labor that goes into making a dataset.
Since we are a team that fundamentally believes in the value of visualizing and analyzing during research and development in AI, we decided to make it possible to take a gander at some of these datasets for CVPR 2023 attendees in Vancouver. Over the last couple weeks, our team at Voxel51 added a handful of these datasets to FiftyOne; today, we are publishing it live at cvpr.fiftyone.ai. If you’re here in Vancouver in person, stop by Voxel51 Booth 1618 to browse in person.
We are intensely grateful to the authors of the datasets who made their work easily accessible and hence includable in cvpr.fiftyone.ai.
How We Found The Datasets
So, how did we find the 59 new datasets as part of CVPR’s publications? Further, how did we decide which datasets would get uploaded into cvpr.fiftyone.ai? First, we got the list of all 2,359 accepted conference papers. Once we had access to them, we looked into the abstracts of each paper to quickly determine if their paper involved creating and/or using a new dataset. Upon searching all of the papers this way, we found 59 papers that suggested they did. Although we did this manually without the help of an LLM, we acknowledge we may have missed some.
From here, we proceeded to look up information on each dataset including but not limited to license information, dataset accessibility, and dataset type (image, video, mesh, etc.). This process allowed us to prune this list of 59 down to a manageable set of 20 or so compatible datasets from which we ultimately added 9. Some of this reduction is due to the classes of datasets that FiftyOne supports, e.g. whereas it supports images, videos, and point cloud, it does not support meshes. Let us explain more.
While researching each of these datasets to upload, we looked at licensing as well as dataset availability. What was surprising to us was that while many of these datasets were marked as available and licensed to use, we were required to get permission from the authors to even download them. This may have been in the form of a Google form, requesting permission from a website to execute download, or even sending an email in some cases clearly outlining the way the dataset was to be used. Going through these datasets, we could only begin working with 5 that were licensed properly, given this is a research conference and available to download immediately. This led us to the question:
How is it possible that out of 2,359 papers at CVPR 2023 and out of the 59 papers that include new datasets to share with the community, we could only access 5 of them?
Following this realization, we followed up with authors on the other datasets that are compatible with FiftyOne. While many of them responded (and we were able to add several more datasets because of this), it slowed us down. Clearly, if you want your dataset easily adopted by the community, make it easily and openly accessible. Not a new lesson, but one that seems to have fallen flat in many cases.
In the following section, you’ll learn a little more about each of the 9 datasets we were able to include in cvpr.fiftyone.ai. If you enjoy browsing these datasets, be sure to thank the authors who made their data so readily available to us that we could provide this opportunity to you! If you enjoy working with FiftyOne as a computer vision and AI research framework, you can thank us by giving us a star on GitHub.
Further, if you find yourself publishing a dataset in the future, consider the benefits to making that data readily available for download with the publication! Add it to the FiftyOne Dataset Zoo.
The Datasets Included in cvpr.fiftyone.ai
Listed below are the datasets we’ve ingested into our FiftyOne Teams database to make it easy to explore. Our team of engineers here worked hard to make the data interesting to visualize, so read below on what each dataset contains and the best ways to start exploring it on your own!
Spring
The Spring dataset comes from the paper Spring: A High-Resolution High-Detail Dataset and Benchmark for Scene Flow, Optical Flow, and Stereo by Lukas Mehl et al. This computer-generated dataset serves as a benchmark for scene flow, optical flow, and stereo data, providing state-of-the-art visual effects within the realistic HD datasets. This dataset is 60x larger than the only scene flow benchmark – KITTI 2015 (also available to browse in cvpr.fiftyone.ai).
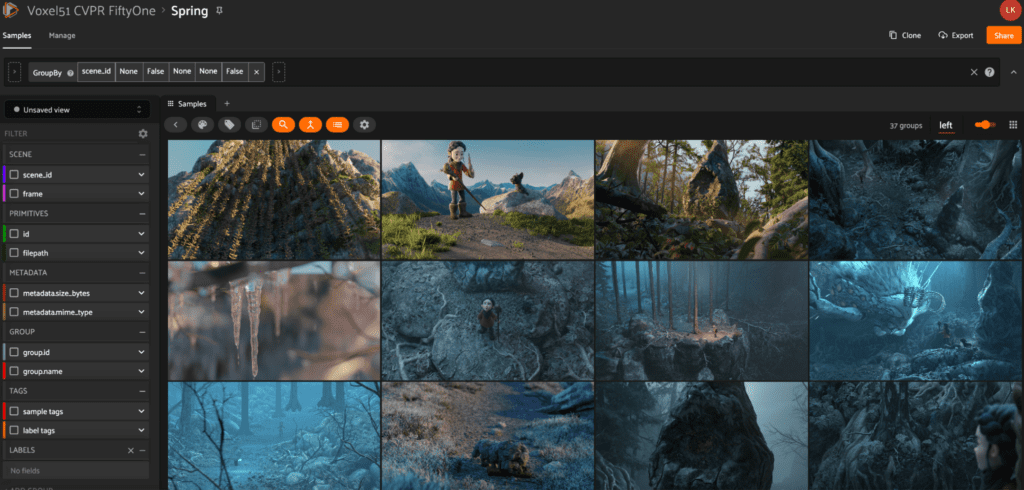
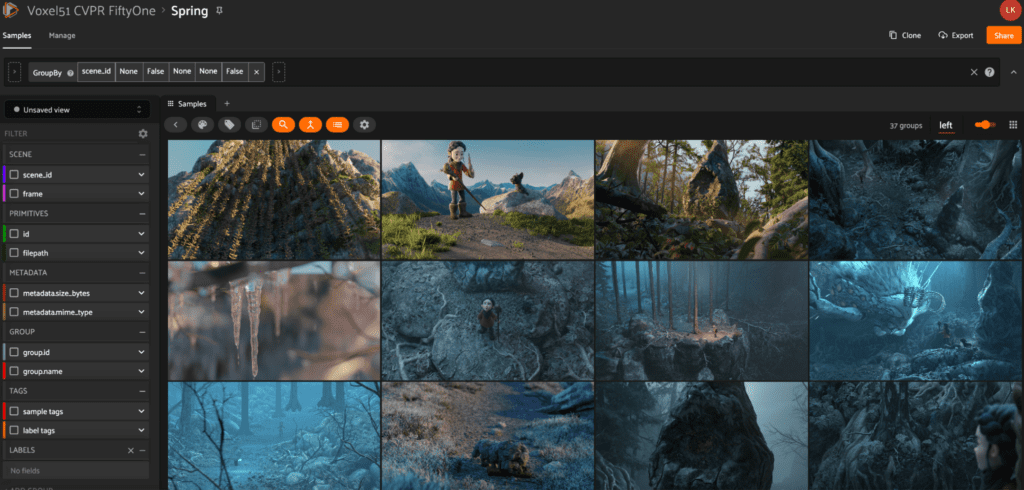
Within cvpr.fiftyone.ai, you’ll find Spring loaded in as groups of images ready to browse by image, providing you with the opportunity to view each image with all of its associated data.
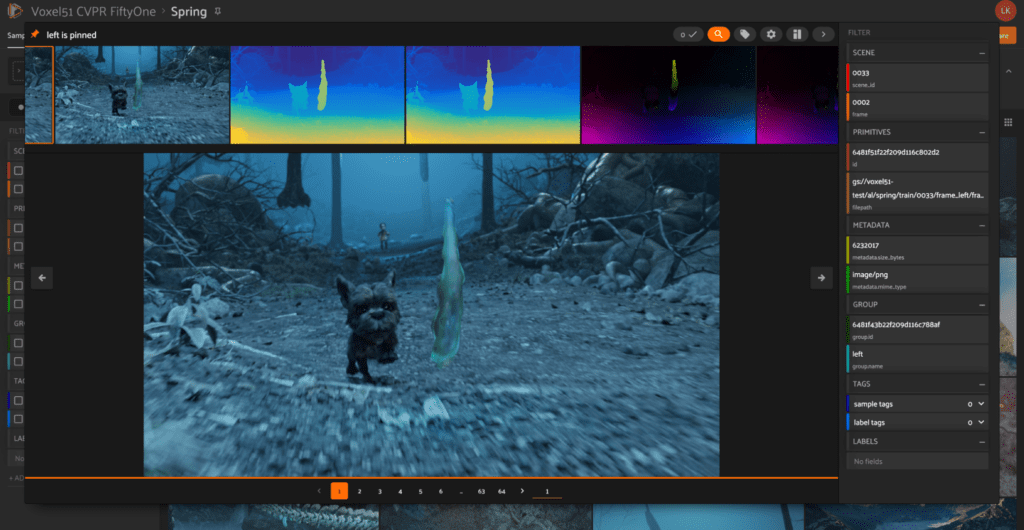
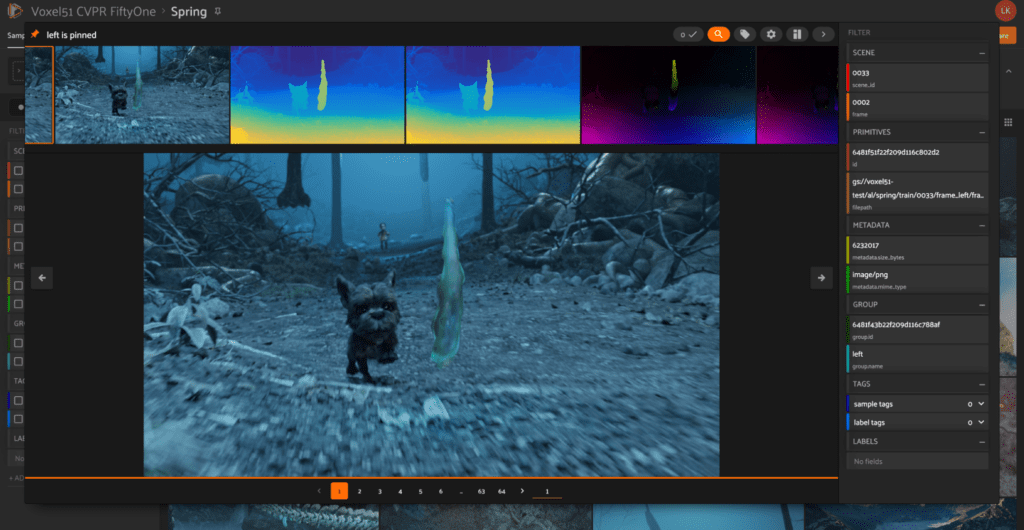
Where to find Spring at CVPR: Tuesday afternoon, Poster #80
GeoNet
The GeoNet dataset comes from the paper GeoNet: Benchmarking Unsupervised Adaptation across Geographies by Tarun Kalluri et al. GeoNet strives to improve machine learning models by providing a dataset that is more inclusive of under-represented locations in the world. To accomplish this, the dataset contains benchmarks to represent diverse tasks such as scene recognition, image classification, and universal adaptation. This new large-scale dataset is curated from existing datasets by selecting images from Asia and the US and separating them into two domains.
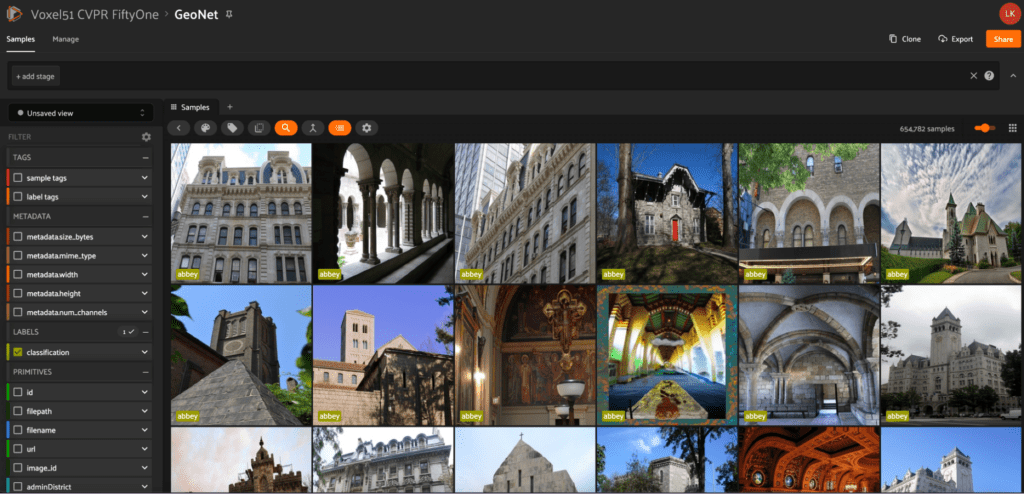
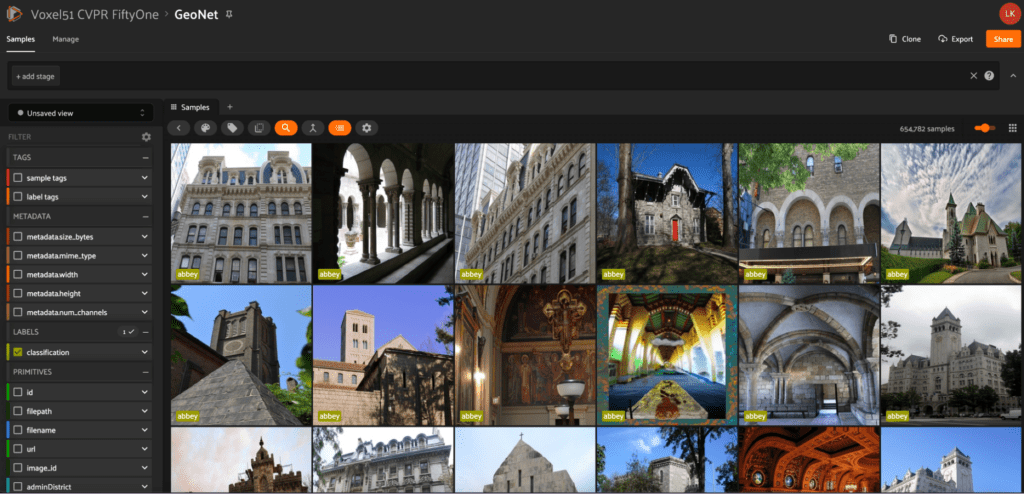
With cvpr.fiftyone.ai, use the map panel to explore images by region and get a better feel for what is being represented in each location!
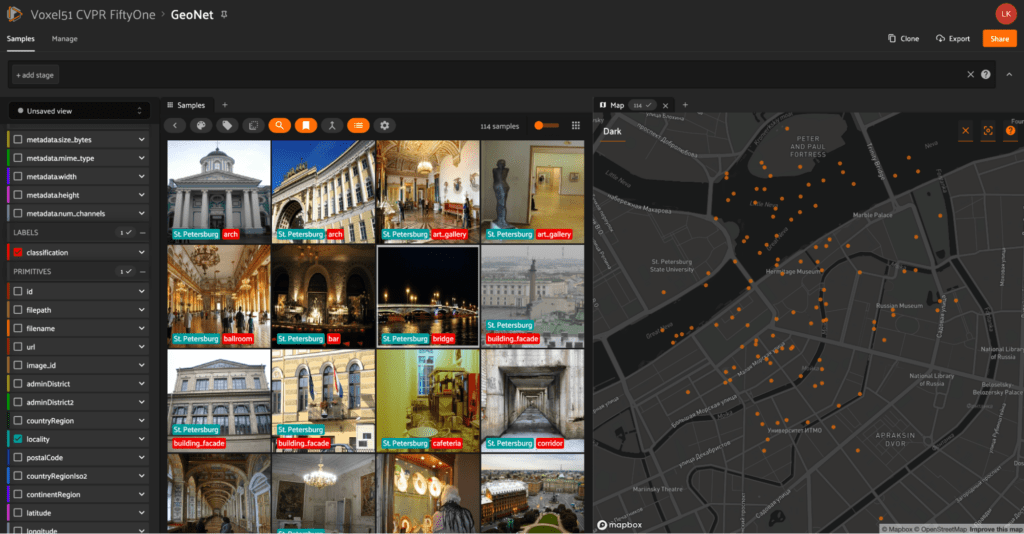
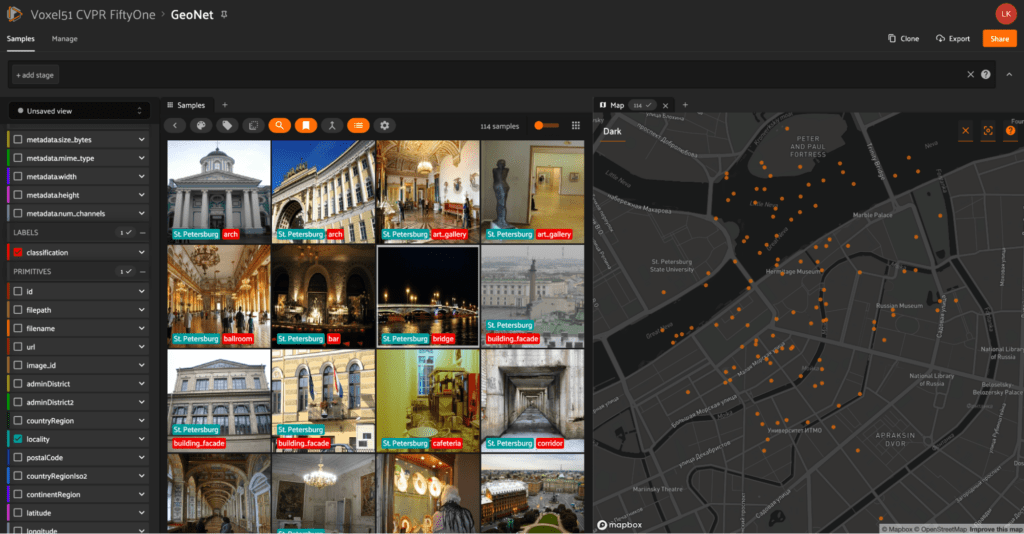
Where to find GeoNet at CVPR: Wednesday afternoon, Poster #287
Mobile-HDR
The Mobile-HDR dataset comes from the paper Joint HDR Denoising and Fusion: A Real-World Mobile HDR Image Dataset by Shuaizheng Liu et al (linked from GitHub) Using three mobile phones and varying lighting conditions, the authors developed the first HDR image dataset captured by using mobile phone cameras (instead of the typical DSLR cameras used in the daytime to capture images in existing HDR datasets). Their experiments showed that this approach provided an advantage over datasets captured with cameras not on mobile phones.
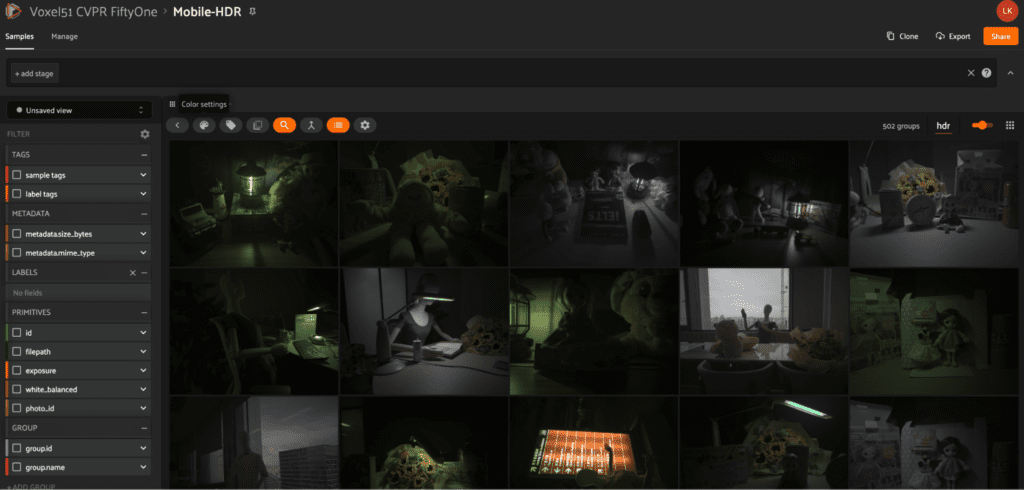
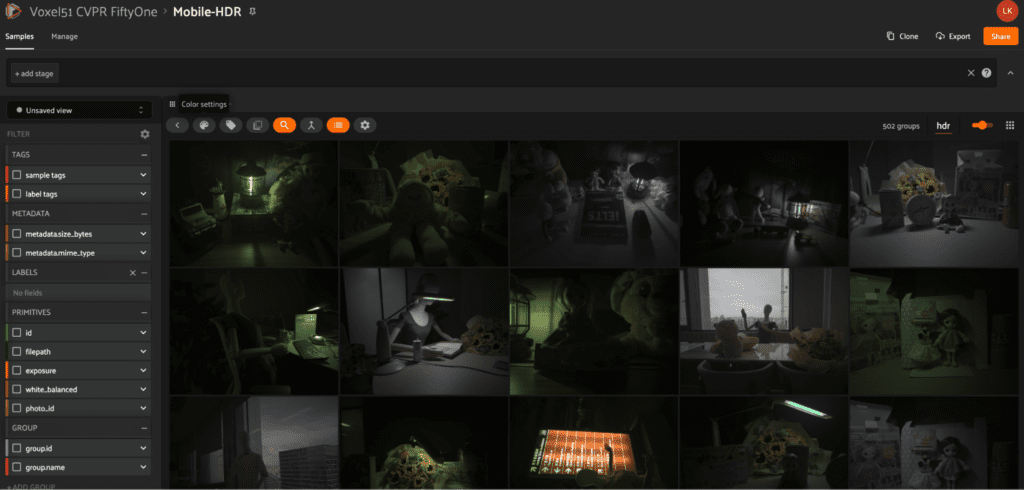
With cvpr.fiftyone.ai, explore images with various camera settings and from different mobile devices!
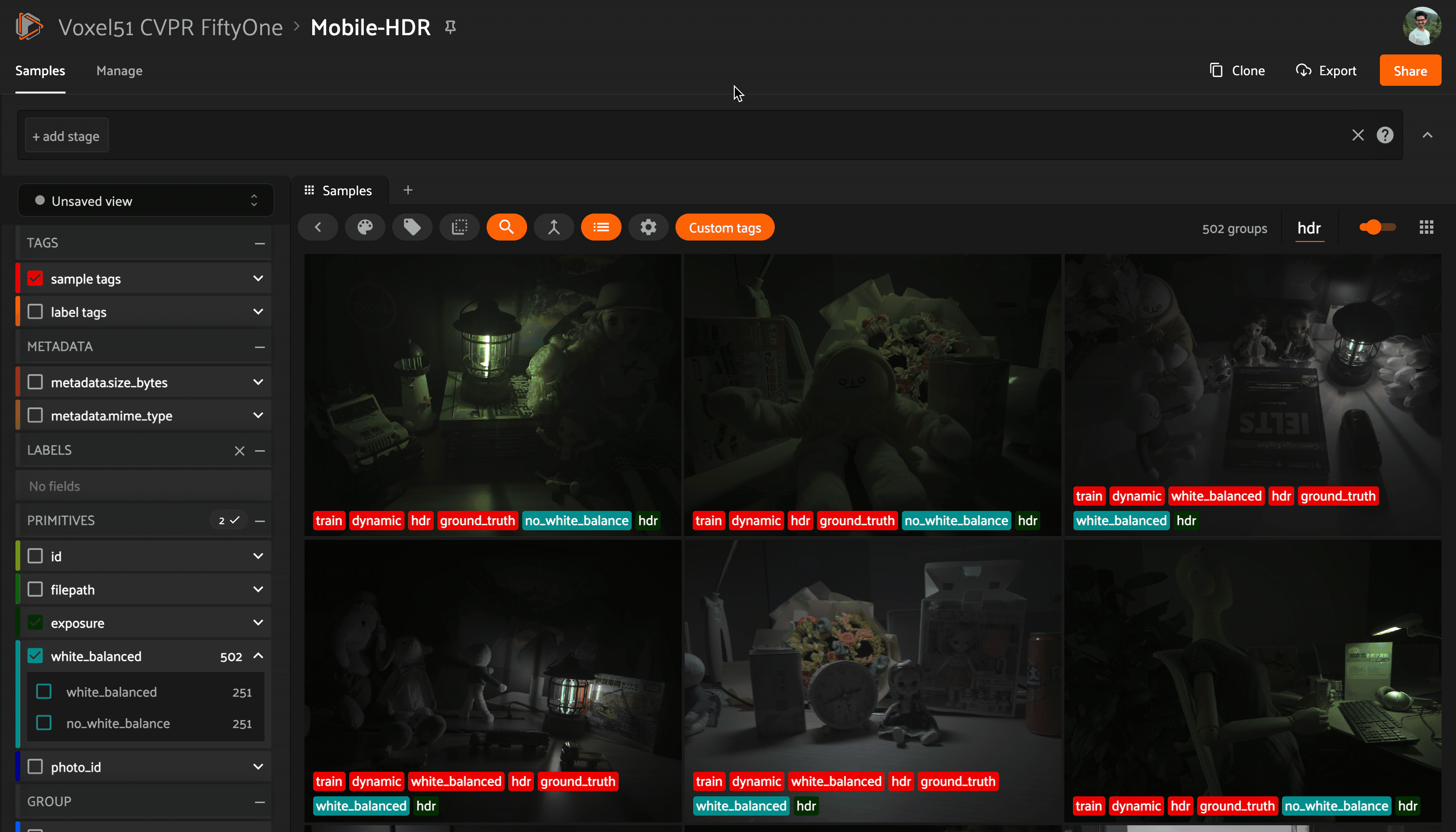
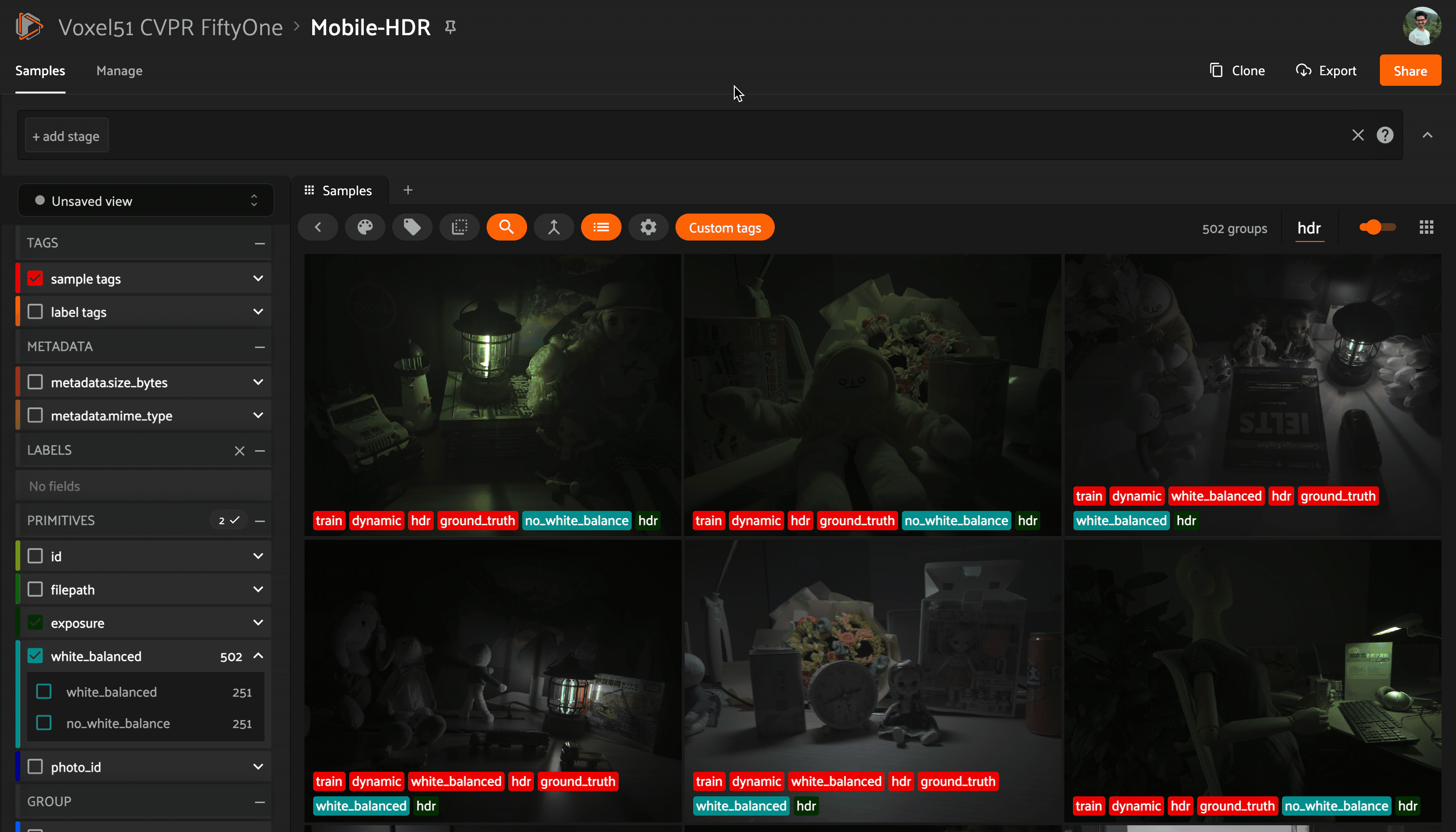
Where to find Mobile-HDR at CVPR: Wednesday afternoon, Poster #154
MVImgNet
The MVImgNet dataset comes from the paper MVImgNet: A Large-scale Dataset of Multi-view Images by Xianggang Yu et al. MVImgNet aims to fill the need for an ImgNet-type dataset for 3D vision by providing a large-scale dataset of multi-view images. The full dataset contains 6.5 million frames across 219,188 videos and covers 238 unique classes. With the inclusion of object masks, camera parameters, and point clouds, MVImgNet provides a soft bridge between 2D and 3D computer vision tasks.
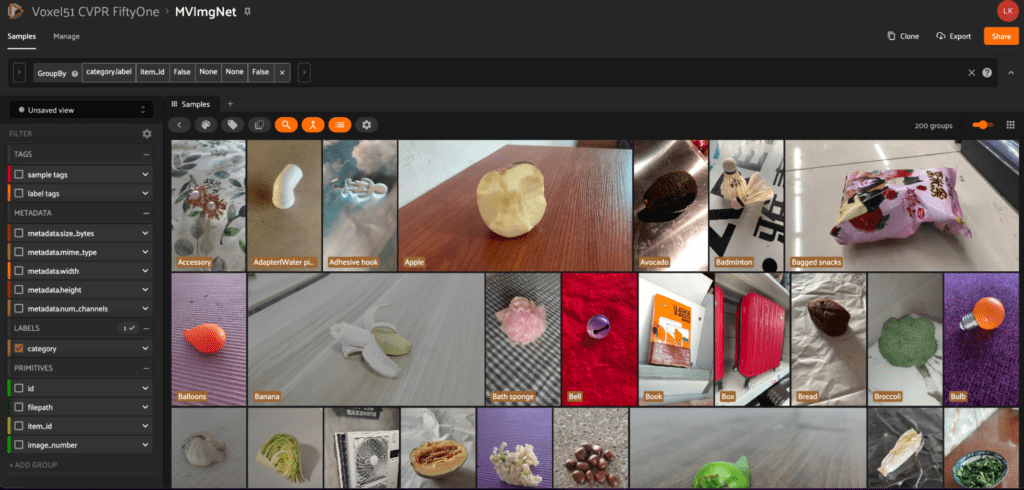
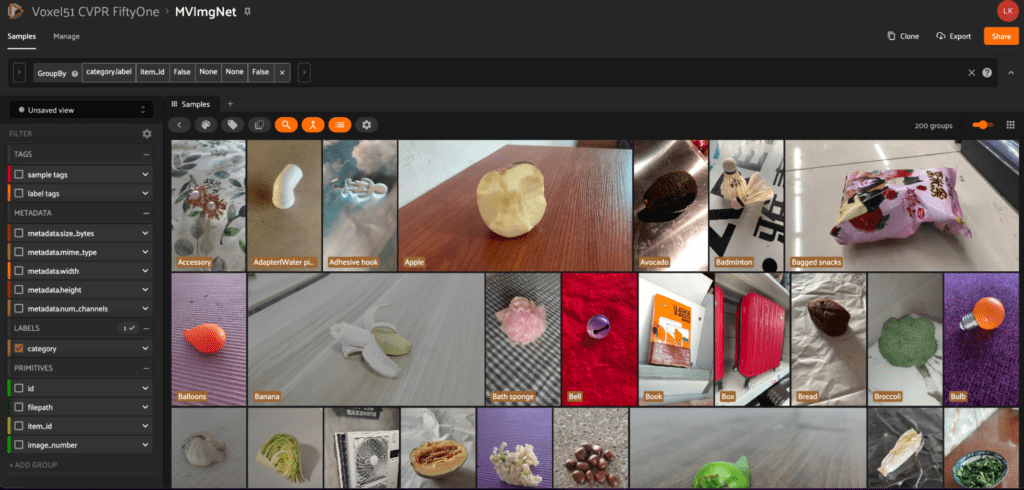
With cvpr.fiftyone.ai, explore the various views of each object, just like you see with this fashionable clutch on a purple background!
Where to find MVImgNet at CVPR: Wednesday morning, Poster #88
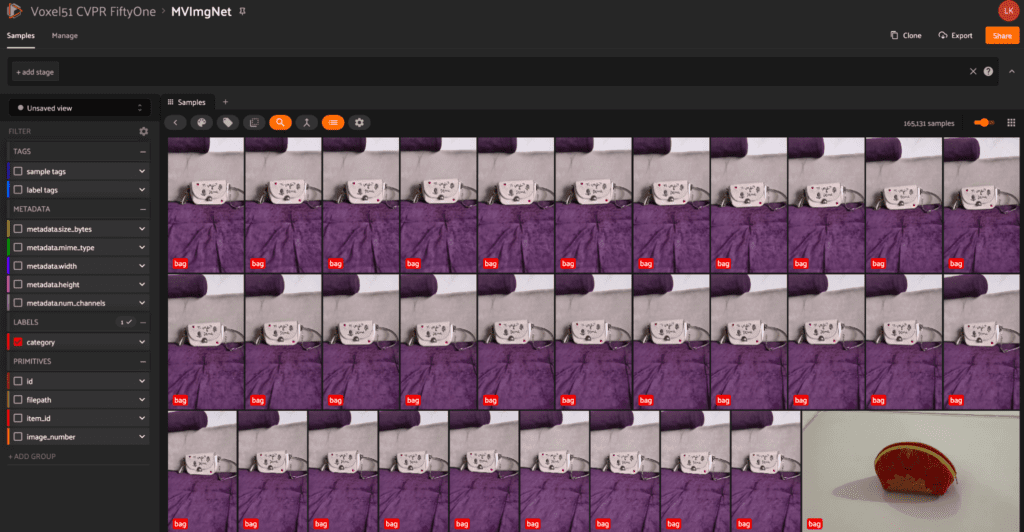
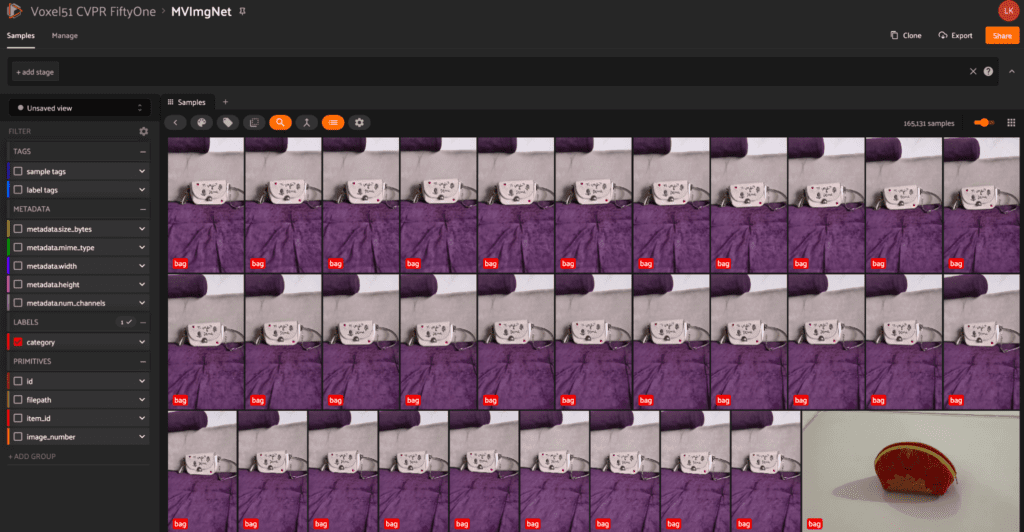
ImageNet-E
The ImageNet-E dataset comes from the paper ImageNet-E: Benchmarking Neural Network Robustness via Attribute Editing by Xiaodan Li et al. This dataset was created using the authors’ toolkit for object editing, controlling backgrounds, sizes, positions, and directions. The goal of this was to create a rigorous ImageNet benchmark (ImageNet-E) for evaluating image classifier robustness in terms of object attributes. In their research, they noticed that even a small change in background led to an average of 9.23% drop in accuracy of top-1 classification.
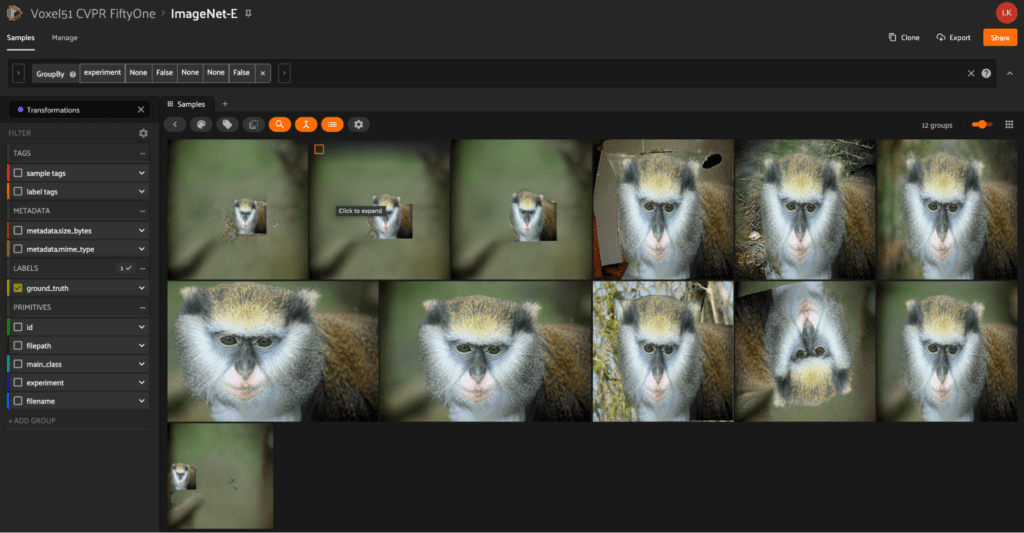
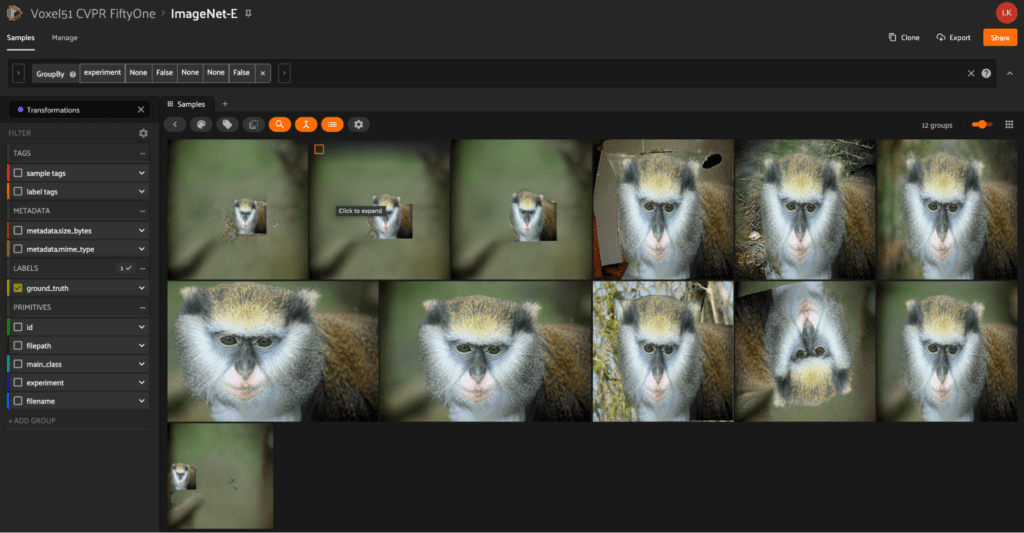
To build their dataset, they selected the animal classes from ImageNet, leading to a total of 4,352 original images. They chose this subset of ImageNet because the animals tend to appear in nature without messy backgrounds. From here, they applied 11 separate transformations on each image, yielding 47,872 total images in the dataset.
With cvpr.fiftyone.ai, use the saved views to explore images by their transformation, classification, or image.
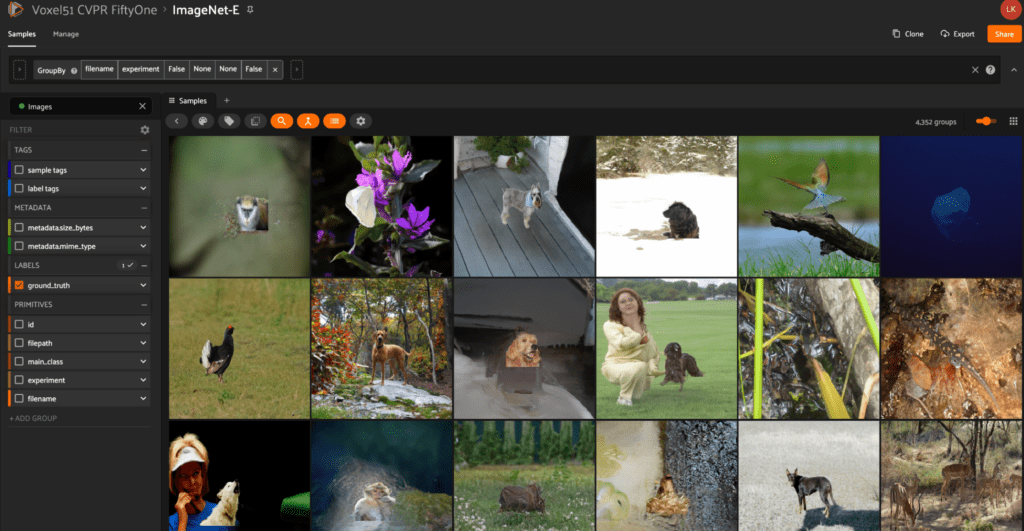
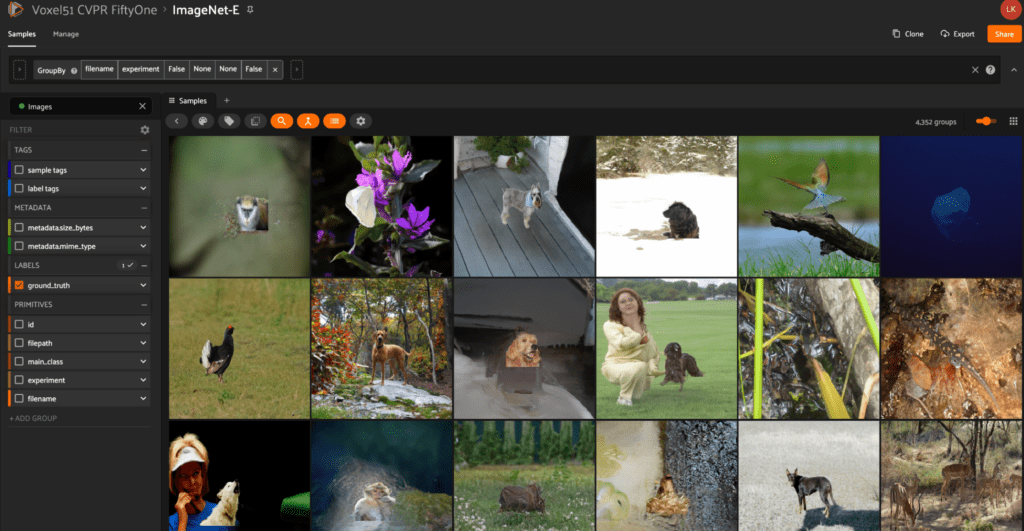
Where to find ImageNet-E at CVPR: Thursday morning, Poster #370
JRDB-Pose
The JRDB-Pose dataset comes from the paper JRDB-Pose: A Large-scale Dataset for Multi-Person Pose Estimation and Tracking by Edward Vendrow et al. Captured from the social navigation robot JackRabbot, JRDB-Pose consists of challenging scenes with crowded indoor and outdoor locations with varying occlusion types. Importantly, the dataset contains human pose annotations with per-keypoint occlusion labels and track IDs consistent across a given scene.
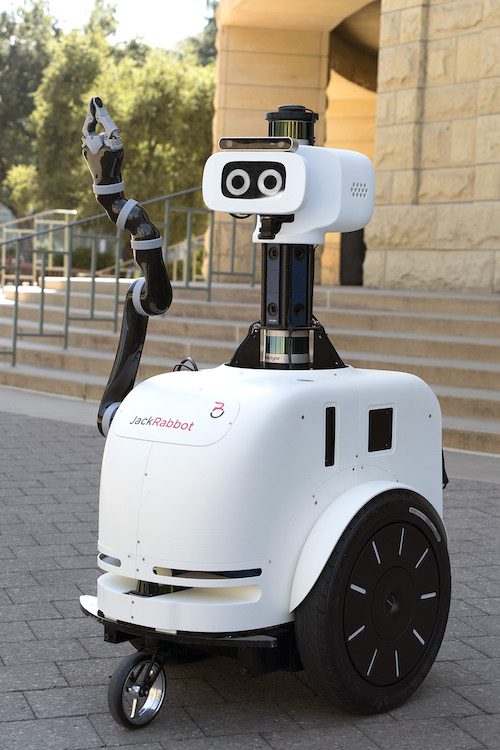
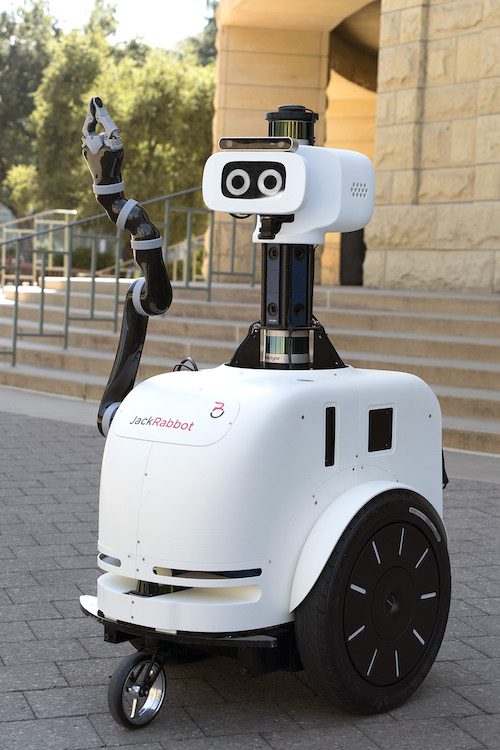
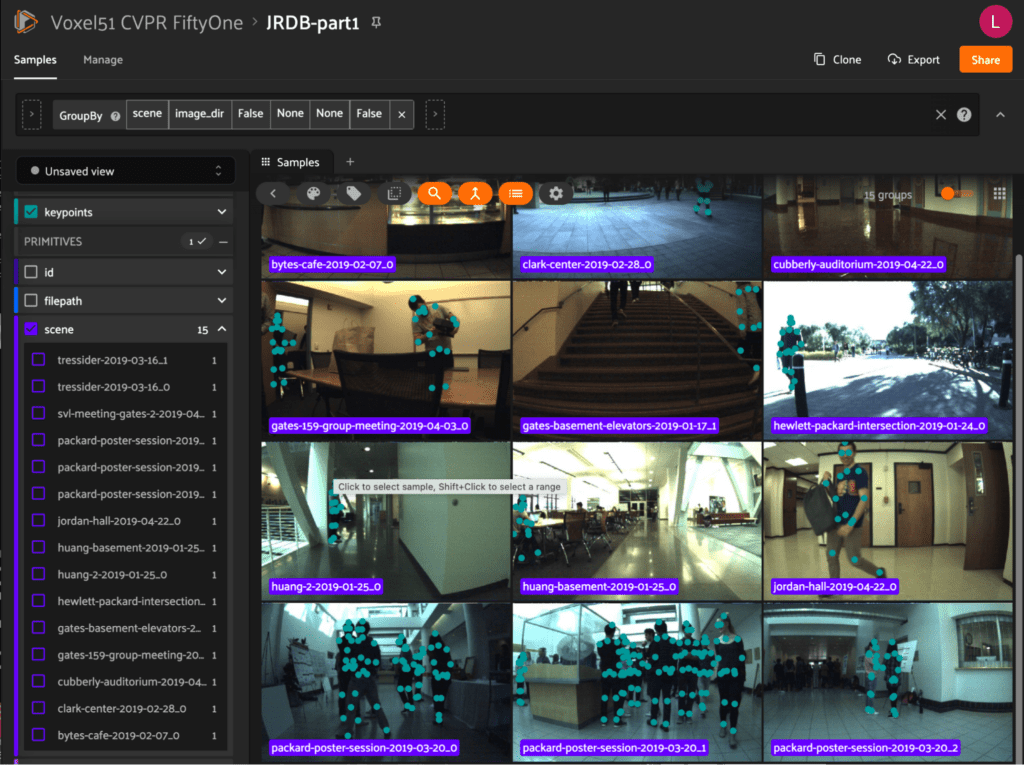
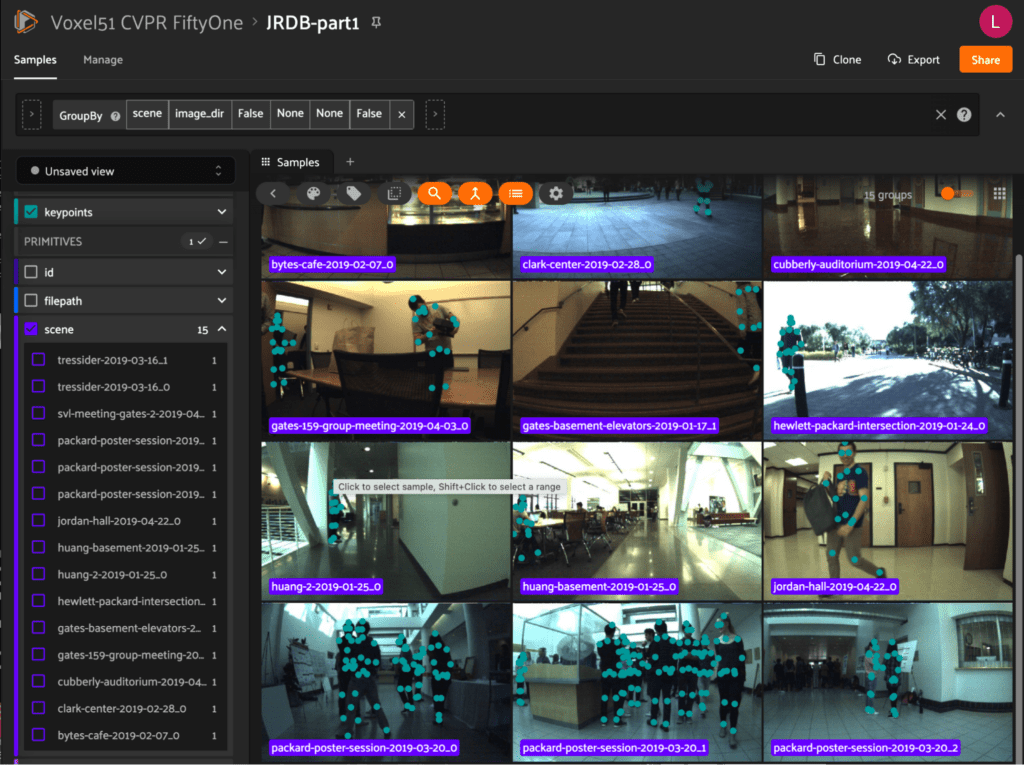
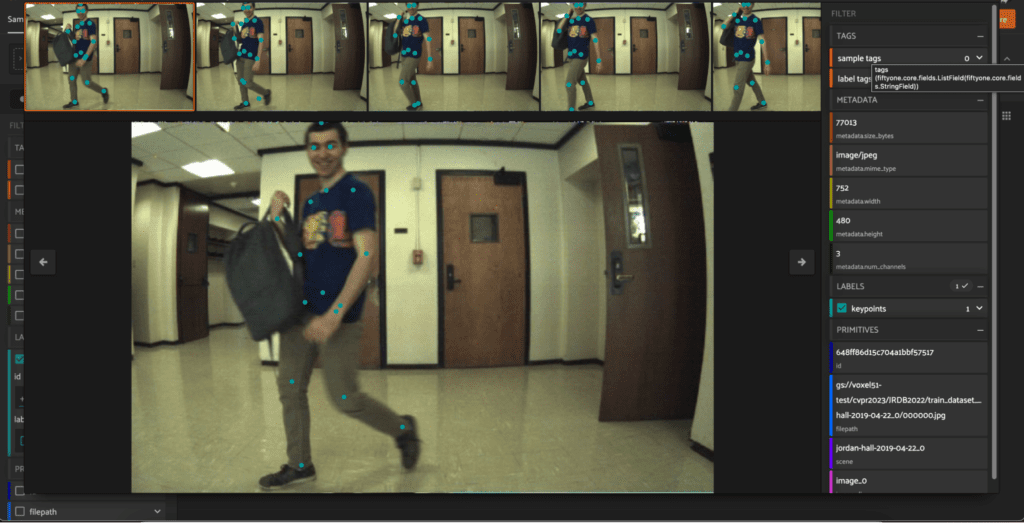
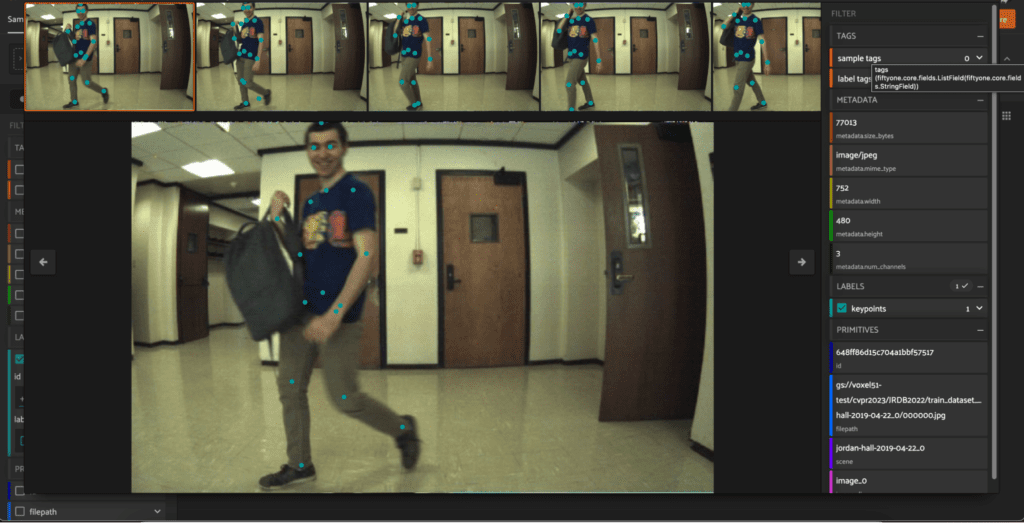
Where to find JRDB-Post at CVPR: Tuesday afternoon, Poster #64. Plus, explore it instantly at cvpr.fiftyone.ai!
ARKitTrack
The ARKitTrack dataset comes from the paper ARKitTrack: A New Diverse Dataset for Tracking Using Mobile RGB-D Data by Haojie Zhao et al. The purpose of ARKitTrack is to provide a new RGB-D tracking dataset for static and dynamic scenes captured with consumer-grade LiDAR scanners. The dataset comes equipped with bounding box annotations, frame-level attributes, and pixel-level target masks.
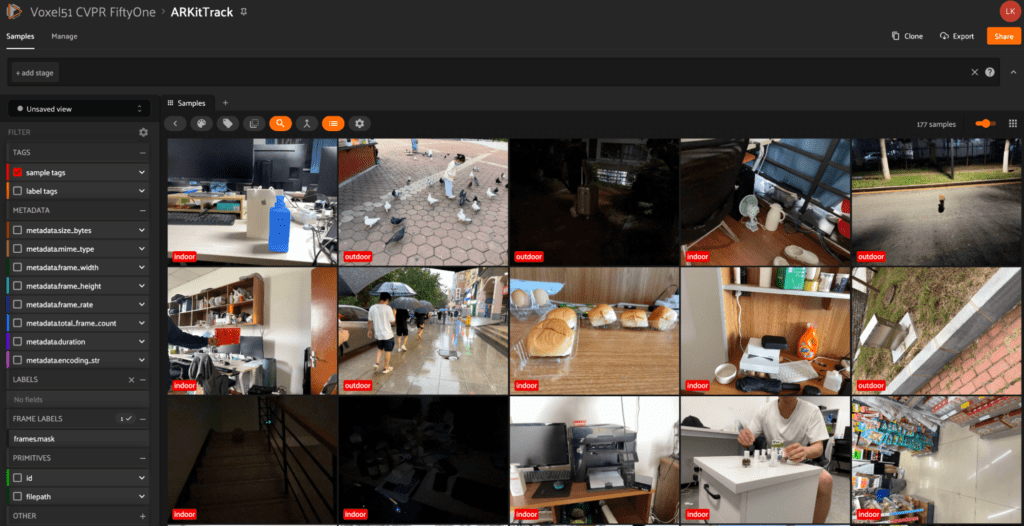
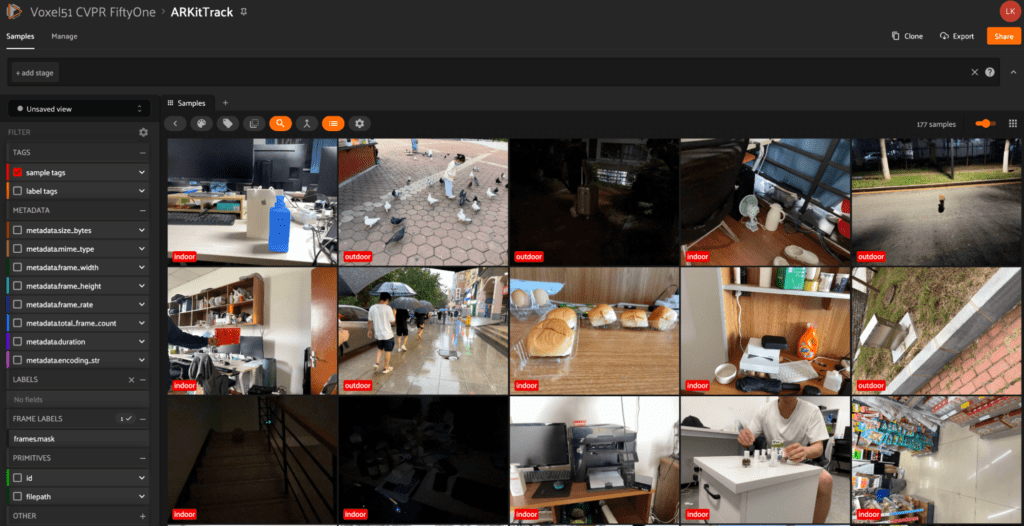
With cvpr.fiftyone.ai, watch the videos and check out the pixel-level target masks!
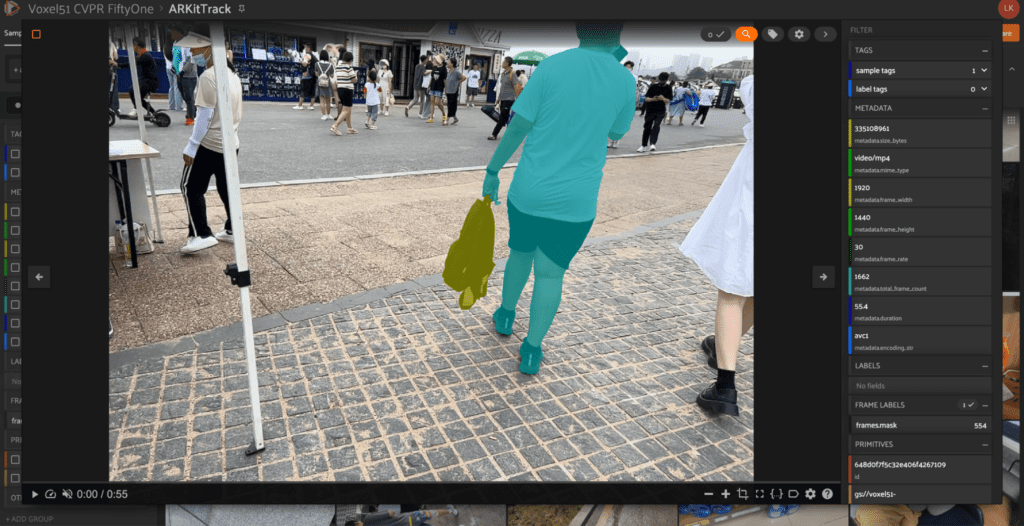
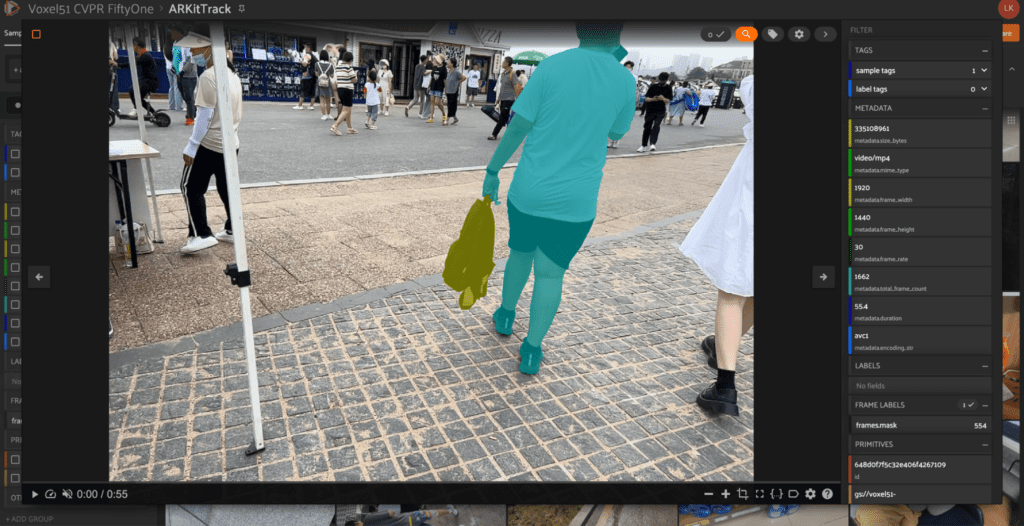
Where to find ARKitTrack at CVPR: Tuesday afternoon, Poster #94
LLCM
The LLCM dataset comes from the paper Diverse Embedding Expansion Network and Low-Light Cross-Modality Benchmark for Visible-Infrared Person Re-identification by Yukang Zhang and Hanzi Wang. LLCM aims to capture low-light cross-modality images of different people captured across 9 different RGB (visible) and IR (infrared) cameras. This dataset was curated in order to more effectively benchmark the authors’ novel augmentation network DEEN (diverse embedding expansion network) against other state-of-the-art methods.
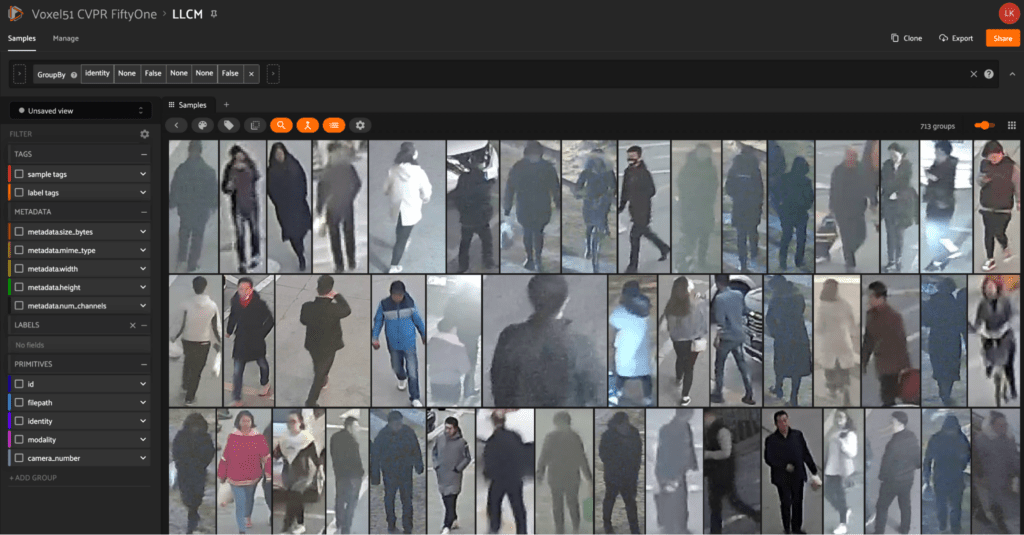
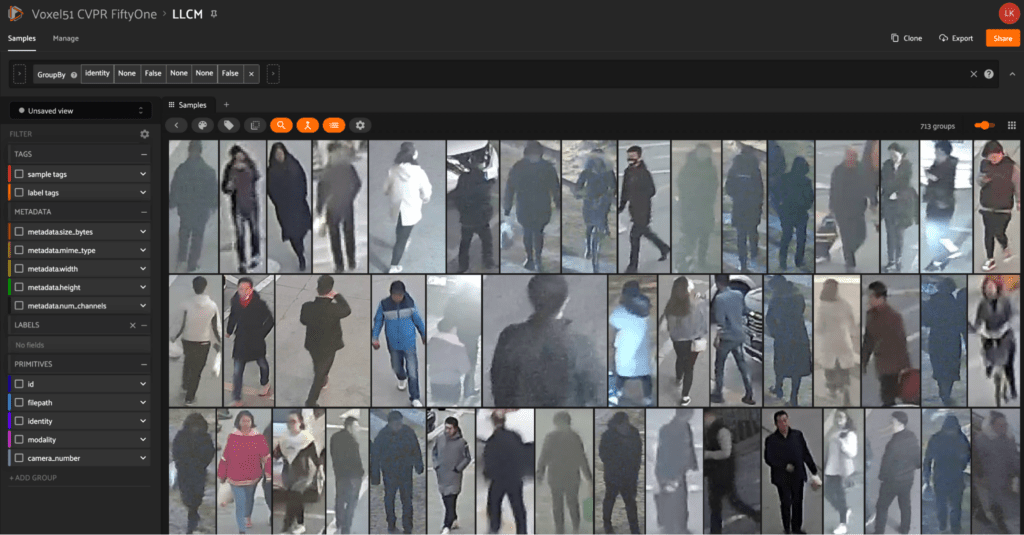
With cvpr.fiftyone.ai, use groups to explore images by each individual and see the different images captured by the various RGB and IR cameras.
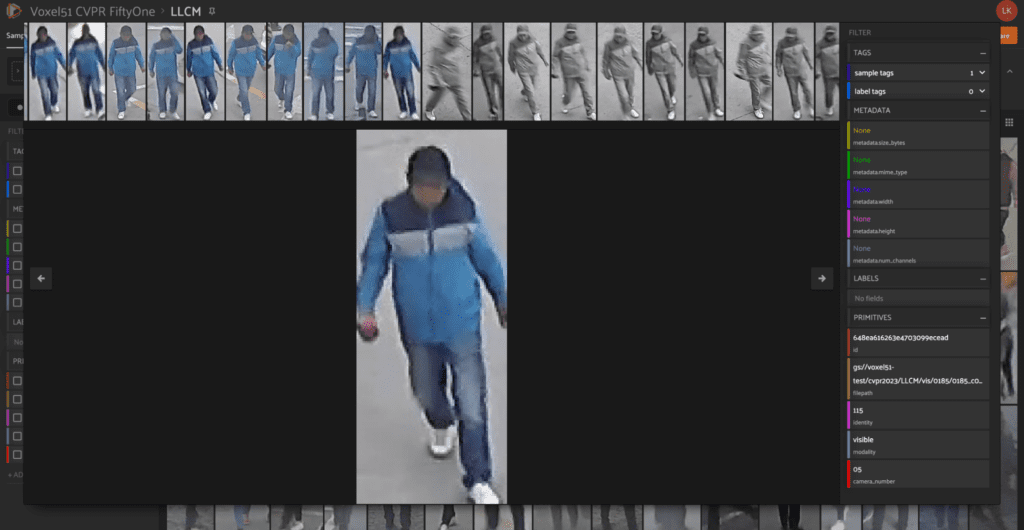
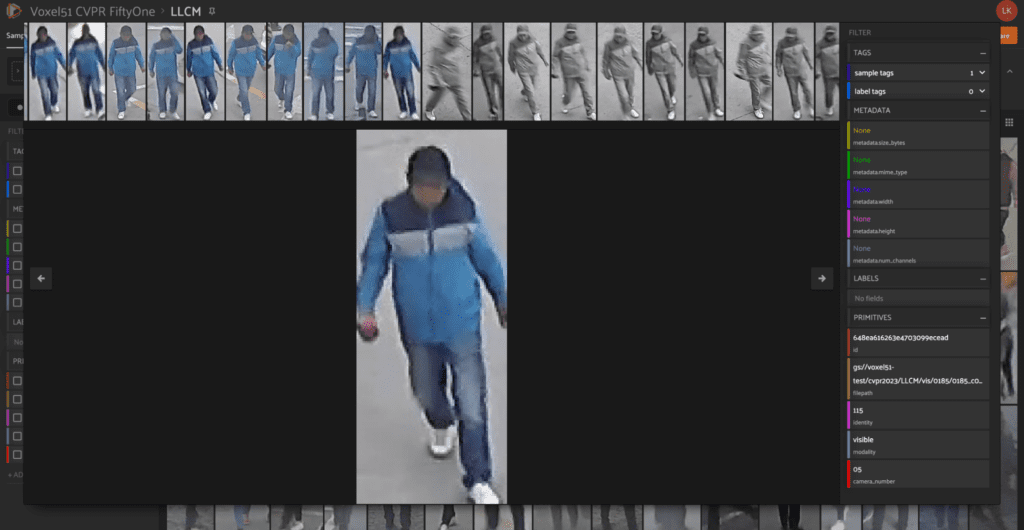
Where to find LLCM at CVPR: Tuesday morning, Poster #206
SynSL-120K
The SynSL-120K dataset comes from the paper A New Benchmark: On the Utility of Synthetic Data with Blender for Bare Supervised Learning and Downstream Domain Adaptation by Hui Tang and Kui Jia. This dataset consists of 120,000 synthetic images across 10 classes. The authors published this dataset as part of their work on bare supervised learning and downstream domain adaptation. The goal of this work was to present the first comprehensive study on synthetic data learning and demonstrate value to the transfer learning community as a new benchmark.
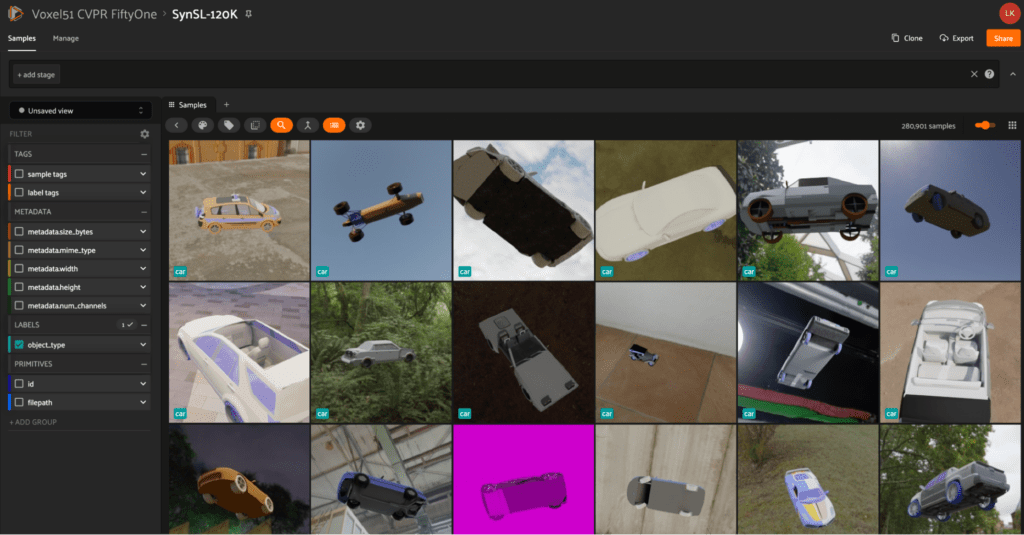
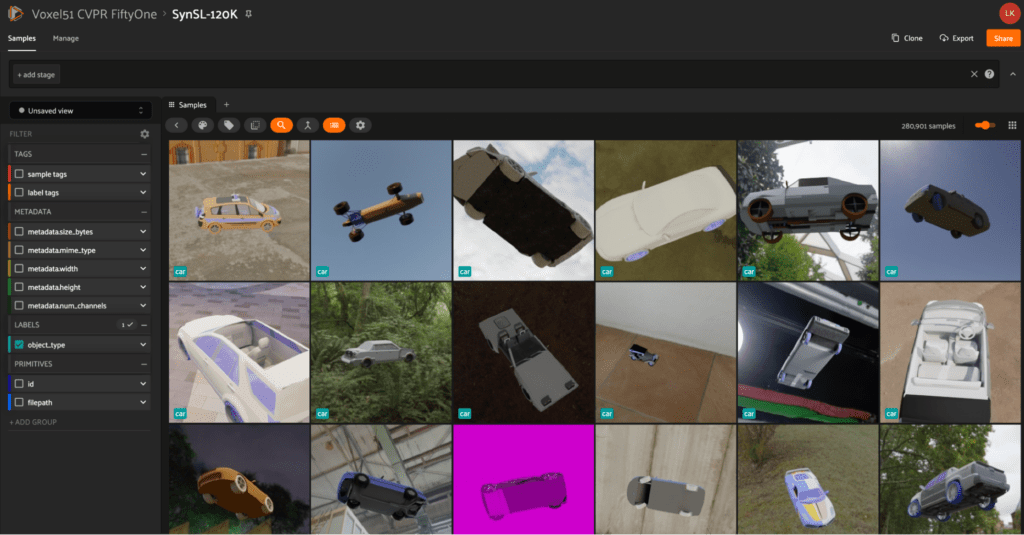
Where to find SynSL-120K at CVPR: Wednesday afternoon, Poster #343
Other Datasets
For those who are less interested in the latest and greatest CVPR data, you can also view a hand-curated selection of dozens of datasets from the FiftyOne Dataset Zoo. But just because it isn’t new doesn’t mean it isn’t exciting—look closely enough and you’ll find the cat wearing a tie and the llama eating a carrot!
Missed Out? Get Your Datasets into the Dataset Zoo Today!
Don’t see your CVPR dataset in the dataset zoo? Want it to be accessible by the entire community? Great news—we’ve made it simple for you to get your dataset into the FiftyOne Dataset Zoo! First, you can test it out locally by adding it to your local dataset zoo. Once you have it how you want it, you can submit a PR to the FiftyOne GitHub repo for your dataset to be included with the next release. Want to follow an example? Check out the PR submitted for the recently added SAMA-COCO dataset!