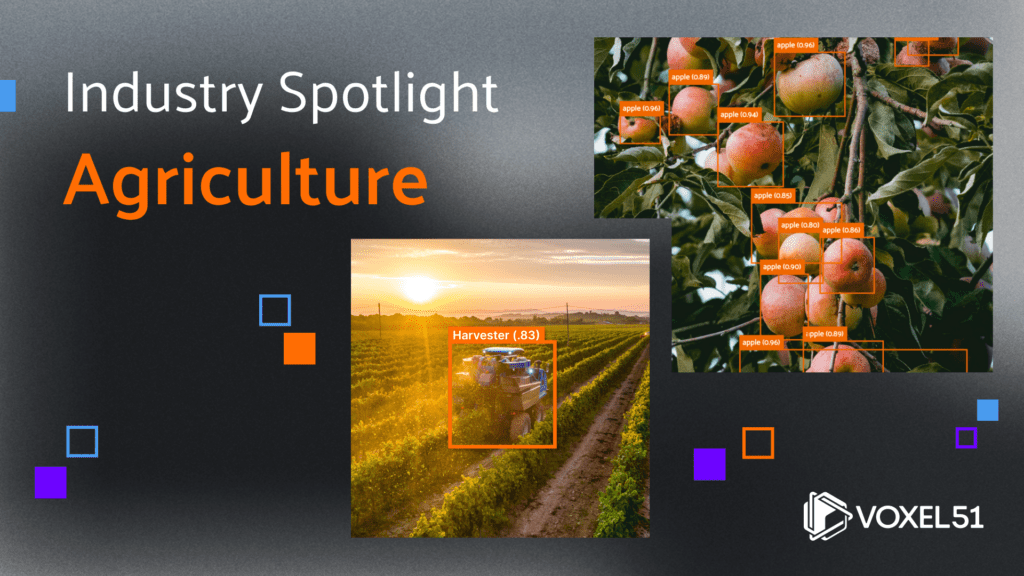
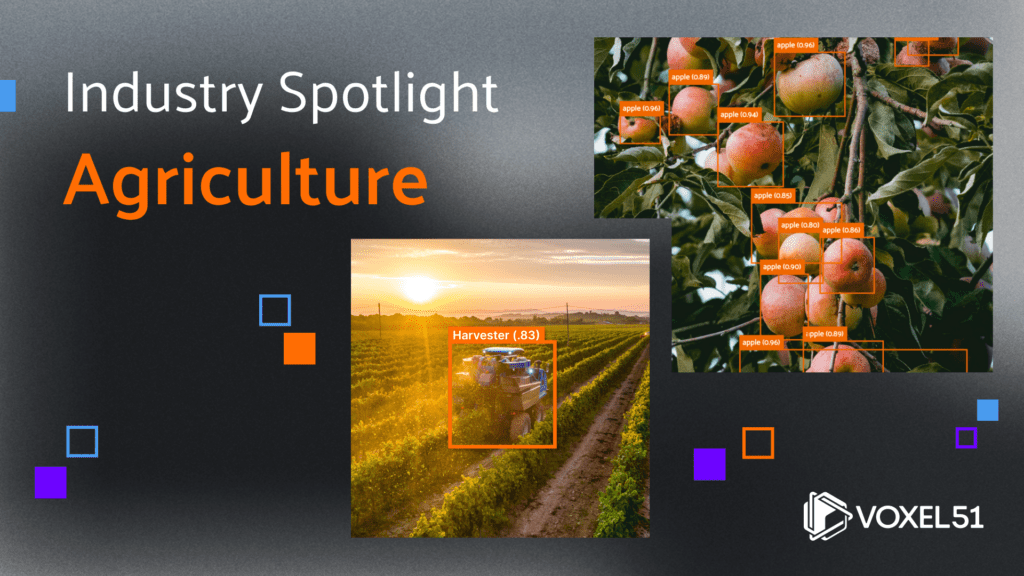
Welcome to the first installment of Voxel51’s computer vision industry spotlight blog series. Each month, we will highlight how different industries – from construction to climate tech, from retail to robotics, and more – are using computer vision, machine learning, and artificial intelligence to drive innovation. We’ll dive deep into the main computer vision tasks being put to use, current and future challenges, and companies at the forefront.
In this inaugural edition, we’ll focus on agriculture! Read on to learn about computer vision in agriculture.
Industry overview
The agricultural industry is ripe for new computer vision-based AI applications. The world’s farmers are tasked with feeding the planet by growing healthier and more productive food, feed, fiber to feed, while also taking care of their land and resources. As in other industries, organizations in agriculture use computer vision and AI applications to drive new innovations and unlock new efficiencies to help them achieve their goals against a backdrop of modern challenges. Before we dive into several popular applications of computer vision-based AI technologies in agriculture, here are some of the industry’s challenges where CV and AI can help.
Key industry challenges in agriculture
- A growing worldwide population: The number of humans is expected to reach 9.8 billion by 2050, leading to dramatic increases in food demand.
- A reduction in arable land: The amount of arable land on Earth is shrinking, with some studies suggesting that farmable land could be halved in the next quarter century.
- A shrinking workforce: The number of people working in agriculture is falling, from 40% of global workers in 2000 to just 27% of global workers in 2019. Labor shortages can result in skeleton crews overseeing hundreds of thousands of acres.
- An increase in climate-related disruptions: The increasing frequency of extreme weather events is expected to lead to decreased crop productivity.
- Pesky pests: According to the Food and Agriculture Organization (FAO), up to 40% of all crops worldwide are lost due to pests. Damages from plant diseases alone total $220 billion per year.
In other words, the agriculture industry will need to feed far more people with fewer resources in a complex and changing environment. The invention and adoption of new technologies will be crucial to overcoming these challenges. AI in agriculture is already valued at more than $1 billion annually, and with a compound annual growth rate (CAGR) of 20%, it is projected to reach $2.6 billion by 2026. Computer vision applications account for a large portion of this existing market, as well as the majority of its anticipated growth.
Continue reading for some ways computer vision applications are helping organizations in agriculture.
Applications of computer vision in agriculture
Precision agriculture
With escalating prices for pesticides, herbicides, and seeds, precision agriculture is helping farmers reduce their costs and get more from their land. As the name suggests, precision agriculture is all about finer-grained control over existing processes, from the placement of crops, to the constitution of the soil, to the application of chemical agents. Computer vision is coalescing with robotics and other emerging technologies to bring this level of precision to agriculture.
Vision techniques are used in the field to make real-time decisions. Object detection techniques are used to identify and localize individual insects and weeds for the application of pesticide, and herbicide, respectively. Precision control also goes beyond where, to how much: nonlinear regression models based on the coloring, size, and other visual attributes of plants can predict exactly what quantity of each chemical the plant should receive. This means optimizing returns while also conserving resources.
For an overview of computer vision techniques in precision agriculture, see Machine Vision Systems in Precision Agriculture for Crop Farming.
Precision livestock farming
Precision livestock farming, or PLF, aims to gain fine-grained insight into and achieve precise control over processes involving cattle, sheep, and other livestock. PLF can be applied for the purposes of maximizing yield, monitoring or ensuring animal health, or decreasing operational carbon footprint.
In precision livestock farming, computer vision techniques are often used in conjunction with GPS tracking and audio signals to generate insights. Together, these techniques can be used to not only identify and track individual animals, but also to analyze their volume, gait, and activity levels.
Here are just a few of the ways computer vision has been utilized in PLF:
- Kinetic depth sensors for classification and detection of aggressive behavior in pigs
- Optical flow for prediction of feather damage in laying hens
- Remote thermal imaging and night vision technology for improving endangered wildlife resource management
For more information about precision livestock farming, see Image Analysis and Computer Vision Applications in Animal Sciences: An Overview and Exploring the Potential of Precision Livestock Farming Technologies to Help Address Farm Animal Welfare.
Autonomous farm equipment
Autonomous farm equipment is important in enabling fewer farmers to farm more land. Along with advances in robot guidance and control, computer vision is helping farmers automate their operations. Machine learning models for object detection and segmentation are making their way onto tractors and harvesters.
Stay tuned for our upcoming industry spotlight blog post about computer vision in autonomous vehicles!
Crop monitoring
To combat crop loss, farmers use data from suites of soil sensors, localized weather forecasts, and multi-level imagery to remotely monitor large tracts of land. This data can be synthesized into “crop intelligence” allowing farmers to take informed action before it is too late.
On the computer vision side, images from satellites, drones, and high-resolution cameras are used for early disease detection and monitoring, soil condition monitoring, and yield estimation.
Some examples include:
- Automatic plant disease diagnosis using mobile capture devices, applied on a wheat use case
- Deep learning-based detection of seedling development
- Soil color analysis based on a RGB camera and an artificial neural network towards smart irrigation: A pilot study
- Deep Gaussian Process for Crop Yield Prediction Based on Remote Sensing Data
For a more thorough discussion of crop monitoring research and applications, see Computer vision technology in agricultural automation —A review.
Plant phenotyping
Climate change will subject many plants to increased temperatures, higher levels of carbon dioxide, and more variable precipitation. It will also make extreme weather events far more common. Some plants will be better equipped than others to survive and thrive.
Plant phenotyping is the process of identifying and understanding how genetic and environmental factors manifest physically, in a plant’s phenome. Computer vision is becoming an important tool in what is widely recognized as a key to global food security, with non-invasive detection, segmentation, and 3D reconstruction techniques giving researchers detailed information about everything from leaf area to a plant’s nutrient levels and biomass. As an example, segmentation of nuclear magnetic resonance images can be used to map the structure of a plant’s three dimensional root system, which plays an important role in the flow of water and nutrients.
Workshops at ICCV and ECCV in recent years focused on problems in plant phenotyping underscore the computer vision community’s commitment to the topic.
Some papers to get you started include:
- Plant phenotyping: from bean weighing to image analysis
- Future scenarios for plant phenotyping
- Lettuce Height Regression by Single Perspective Sparse Point Cloud
- Metric Learning on Field Scale Sorghum Experiments
Grading and sorting
After produce is plucked from the field, and before it ends up in the fresh food aisle of your local grocery store, it may be subjected to quality control processes. For fruits and vegetables, this takes the form of grading and sorting based on size, shape, color, and other physical characteristics. For grains and beans on the other hand, similar sorting processes are used to detect defects and filter out foreign material.
While grading and sorting were traditionally performed by hand, computer vision is now helping humans with much of this work. Optical sorting uses image processing techniques like object detection, classification, and anomaly detection to incorporate quality control into food production and preparation. By 2027, the optical sorting market is expected to surpass $3.8 billion.
One final batch of papers:
- An automated machine vision based system for fruit sorting and grading
- Computer Vision Based Fruit Grading System for Quality Evaluation of Tomato in Agriculture industry
- Fruits and vegetables quality evaluation using computer vision: A review
- Prospects of Computer Vision Automated Grading and Sorting Systems in Agricultural and Food Products for Quality Evaluation
Companies at the cutting edge of computer vision in agriculture
Carbon Robotics
Founded in 2018 and headquartered in Seattle, agricultural robotics startup Carbon Robotics has raised $35.9 million to help farmers wage war against weeds with lasers, rather than whackers. The company’s LaserWeeder technology uses the thermal energy in 30 onboard lasers to target weeds without harming crops.
The LaserWeeder uses object detection to identify and precisely locate weeds. High resolution images taken by mounted cameras are fed through an onboard Nvidia GPU and predictions, generated in milliseconds, are communicated to the lasers for firing. When attached to a tractor, this implement is able to eliminate 200,000 weeds per hour.
Carbon Robotics’ Autonomous LaserWeeder also uses computer vision techniques, in conjunction with GPS location data, for autonomous navigation. In addition to the weed detection model, this autonomous agent is also equipped with a furrow detection model, which allows it to distinguish the trail it is supposed to follow from plant beds.
The company was a sponsor for ICCV in 2021.
OneSoil
Founded in 2017, Zurich-based OneSoil employs computer vision techniques on satellite imagery to help farmers maximize their yields and reduce costs.
In 2018, OneSoil released the OneSoil Map – a comprehensive map of farmland across 59 countries. To generate this map, they used 250 Tb of satellite imagery shot by the European Union’s Sentinel-2 satellite. Using proprietary computer vision models, they detected clouds, shadows, and snow, and removed these to generate clean images. To combat the low-resolution of satellite images, they combined images taken over a multi-year span.
The free OneSoil App, which was crowned the 2018 Product Hunt AI & Machine Learning Product of the Year, allows farmers to zoom in and select their plot of land without the need to delineate the boundaries themselves. The key to this feature is OneSoil’s field boundary detection model. To train the model, they worked with a number of farmers to get small samples of field boundary data, and used data augmentation operations to increase the size of their training dataset by multiple orders of magnitude. Given the ease of use, it’s no wonder that more than 300,000 farmers use the app, representing around 5% of the world’s arable land.
As of 2023, OneSoil’s computer vision models can identify 12 major crop types, and the company uses this information to generate productivity zone and soil brightness maps which help farmers make better use of their land.
Taranis
Located in Westfield, Indiana, Taranis has been around for almost a decade and raised more than $100 million to bring farmers leaf-level insights. The Taranis team has collected and digitized a dataset consisting of over 50 million submillimeter, high-resolution images, and over 200 million data points. These images are used to train custom computer vision detection algorithms for weeds, diseased plants, insects, and nutrient deficiencies.
Taranis’s AcreForward Intelligence uses real-time imagery from multiple sources, such as drones, planes, and satellites, to identify insect damage on a per-leaf basis, detect weeds before they become a problem, find nutrient deficiencies, and count the number of plants in a field so farmers can make informed decisions about planting and usage of inputs.
In 2022, one of the world’s largest venture capital firms, Andreessen Horowitz, named Taranis one of the top 50 companies kickstarting the American renewal.
Blue River Technology
A subsidiary of John Deere, Blue River Tech was acquired by the agriculture powerhouse in 2017 for a cool $305 million. When the company started, they narrowed in on lettuce farming, using computer vision and machine learning models to help space plants for maximal yield. Since these relatively humble beginnings, Blue River Tech’s computer vision capabilities have expanded to include sensor fusion, object detection, and segmentation.
All of these techniques come together in their See & Spray technology. In traditional broadcast spraying, chemicals are sprayed uniformly over an entire field. This practice leads to wasted herbicide, which is costly to the farmer, can pollute the environment, and can foster resistance to the applied chemicals. See & Spray uses object detection to identify weeds in real time so that herbicide can be applied only where it is needed, resulting in 77% reduction in herbicide.
Blue River Tech takes weed detection further by housing its See & Spray technology in an autonomous tractor equipped with 6 stereo cameras. Together, these cameras allow for an on-board computer to estimate depth information for objects surrounding the tractor using sensor fusion. Color (RGB) data and depth information are fed into a semantic segmentation model, which divides the world into five categories: drivable terrain, sky, trees, large objects such as people, animals, and buildings, and the implement being used by the tractor.
The autonomous tractor stops whenever a large object is detected in its path, and is trained to err on the side of caution by weighing false negative large object detections more strongly than false positives. When the tractor stops, the images are sent to the cloud to be reviewed by humans.
Blue River Tech’s computer vision models were trained on a growing dataset which already contains more than one million images.
Agriculture datasets and competitions
If you are interested in exploring applications of computer vision in agriculture, check out these datasets and competitions:
- Sorghum Biomass Prediction
- Root Segmentation Challenge
- Leaf Segmentation and Counting Challenges
- Global Wheat Dataset and Challenge
- Aerial Sheep Dataset
- DeepWeeds: A Multiclass Weed Species Image Dataset for Deep Learning
- PlantDoc: A Dataset for Visual Plant Disease Detection
For a detailed discussion of openly available datasets for weed control and fruit detection, check out A survey of public datasets for computer vision tasks in precision agriculture.
If you would like to see any of these, or other computer vision agriculture datasets added to the FiftyOne Dataset Zoo, get in touch and we can work together to make this happen!
Join the FiftyOne community!
Developers of agricultural applications can benefit from FiftyOne’s ability to easily filter through the huge amounts of visual data collected daily from farms and other sources. Using open source FiftyOne, this data can be curated into datasets for model training, or to share with experts for annotation or analysis of CV models. Join the thousands of engineers and data scientists already using FiftyOne to solve some of the most challenging problems in computer vision today!
- 1,300+ FiftyOne Slack members
- 2,450+ stars on GitHub
- 2,700+ Meetup members
- Used by 231+ repositories
- 55+ contributors