How Computer Vision Is Changing Retail
February 6, 2024 – Written by Dan Gural , Monica Tran
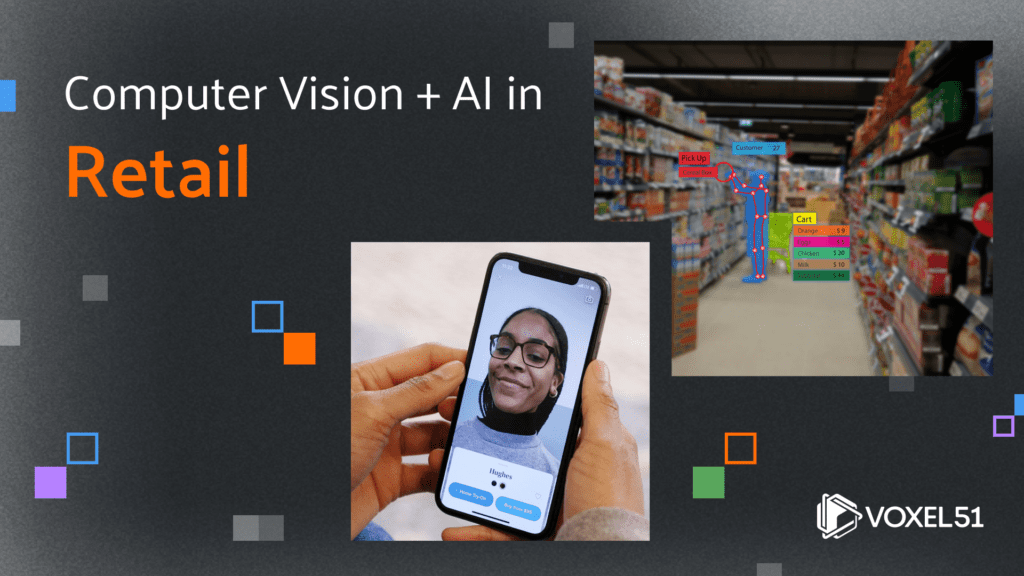
Welcome to the fifth installment of Voxel51’s computer vision industry spotlight blog series. Each edition, we highlight how different industries – from construction to climate tech, from medicine to robotics, and more – are using computer vision, machine learning, and artificial intelligence to drive innovation. We’ll dive deep into the main computer vision tasks being put to use, current and future challenges, and companies at the forefront.
In this edition, we’ll focus on retail! Read on to learn about computer vision in the retail and ecommerce industry.
Retail Industry Overview
Retail is an important pillar of modern economies, bridging the gap between producers and consumers. It’s a sector that facilitates commerce and reflects and shapes societal trends and consumer preferences.
Key facts and figures:
-
- The global retail market size grew from $26.18 trillion in 2022 to $28.34 trillion in 2023, with a Compound Annual Growth Rate (CAGR) of 8.3%.
-
- In the United States, retail sales were expected to grow moderately yet positively between 4% and 6% in 2023.
-
- Ecommerce will continue to grow in popularity, with ~21% of retail purchases expected to take place online in 2023, rising to 24% by 2026.
-
- The value of AI in the retail market is estimated to be around $7.1 billion in 2023, marking a 29% increase from the previous year.
Applying computer vision and AI to retail opens up a whole new world of possibilities. Recent innovations in both retail and retail technologies have set the stage for today’s advancements. Thanks to vision- and AI-powered technologies, retailers can get a better read on what shoppers want, meet those needs more sustainably, and continue making shopping an enjoyable omnichannel affair.
Before we dive into various popular applications of computer vision-based AI technologies in retail, it’s important to highlight the key challenges facing the industry.
Key Industry Challenges in Retail
-
- Supply Chain Issues: Excess inventory has emerged as a significant challenge globally, including extra inventory of high-tech electronics components totaling more than $250 billion in the US alone.
-
- Loss Prevention and Security: The retail sector continues to experience losses due to theft and other criminal activities. In FY 2022, the average shrink rate increased to 1.6% from 1.4% in FY 2021, amounting to $112.1 billion in losses.
-
- Evolving Customer Expectations: According to a report by Zendesk, 73% of consumers will switch to a competitor following multiple bad experiences, indicating a high level of expectation throughout the customer experience.
-
- Evolving Ecommerce & Omnichannel Expectations: Evolving consumer expectations aren’t just limited to brick-and-mortar shopping experiences; they extend to ecommerce and omnichannel experiences, too.
-
- Sustainability Initiatives: Consumers are increasingly holding brands responsible for sustainability, with 46% looking to brands to lead in creating sustainable change.
Continue reading to learn about several ways in which computer vision applications are helping organizations in the retail industry.
Computer Vision Applications in Retail
Inventory Management & Supply Chain Optimization

Inventory management is all about getting the right products to customers at the right place and time, avoiding frustrating stockouts and wasteful overstocking. Computer vision is an ideal ingredient in inventory management systems due to the availability of visual data from cameras and other sensors that make it possible to monitor and analyze inventory levels in real time.
Computer vision techniques at the core of AI-powered inventory management systems include object detection, object recognition, image classification, and more. Visual search is increasing in popularity, enabling consumers to search for products using images instead of (or in addition to) text.
Vision-based inventory management systems bring a variety of benefits to retailers and consumers, including enhanced operational efficiency, increased customer satisfaction, cost savings, and streamlined supply chains. The automation of inventory processes also frees up valuable staff time, allowing workforces to focus on other value-added tasks and continuing to improve the overall shopping experience for customers.
Computer vision is also paving the way for a new suite of AI-powered tools to help retailers optimize inventory management, including smart shelf solutions, AI route optimization for deliveries, store layout optimization, and in-store shopper analytics.
For further reading on the use of computer vision and AI technologies for automated inventory management at popular retailers, visit the following resources:
Additionally, here are a few academic papers related to using computer vision for real-time inventory management:
Autonomous Checkouts & Smart Carts
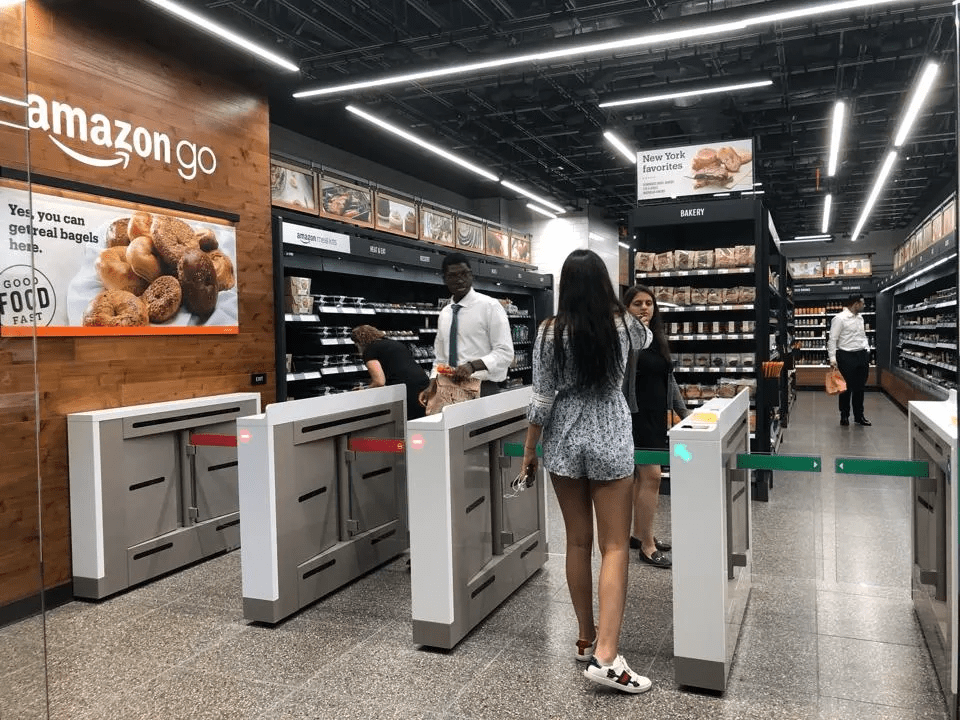
Autonomous checkout systems and smart carts in retail utilize computer vision to deliver a fast, efficient shopping experience. By employing cameras, scanners, sensors, and object recognition concepts, these systems can instantly recognize and tally products. Shoppers can simply place their items in a designated area, and the system automatically calculates the total, facilitating a seamless and rapid checkout experience.
For retailers, self-checkout systems increase checkout speeds, accuracy, and efficiency, while also reducing labor costs, which is especially important in a tight labor market. For shoppers, contactless checkout systems offer a smooth grab-and-go shopping experience, while reducing the time spent at checkout counters and enhancing overall convenience.
Visit these resources for further reading on automated checkout systems at popular retailers:
Check out these papers about using computer vision for automated checkout systems:
Virtual Try-Ons
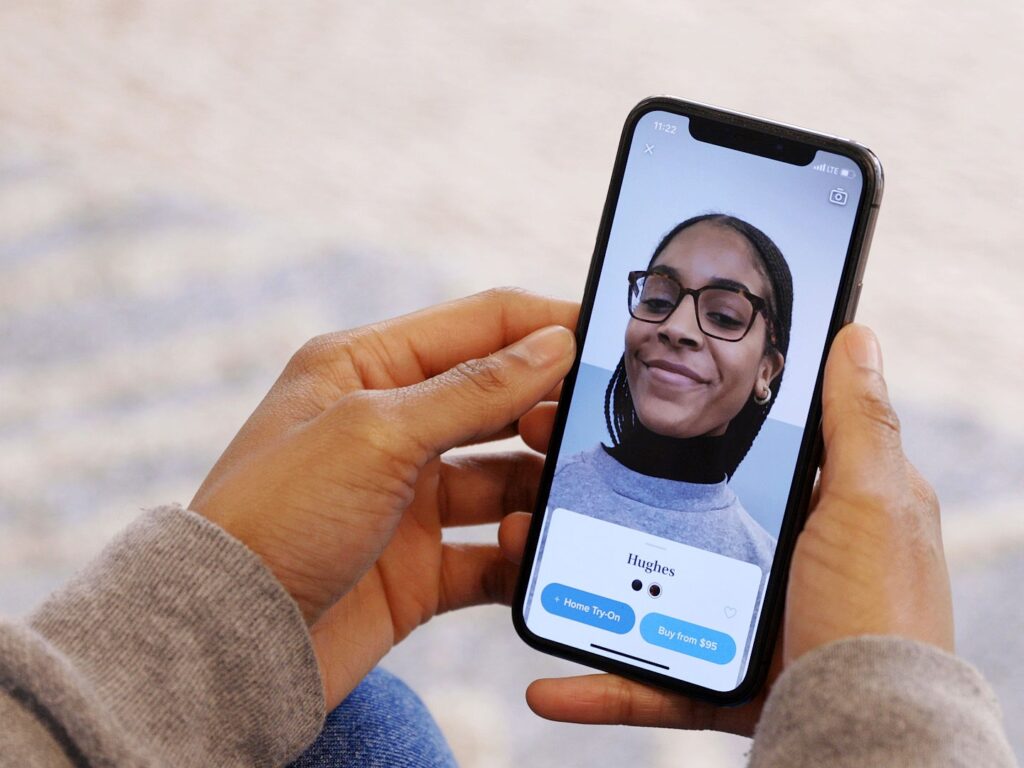
Virtual try-ons in retail allow customers to virtually “wear” clothing, accessories, and makeup from the comfort of their own homes using digital overlays on their images or live feeds. These systems analyze the user’s physical features using pose estimation, image segmentation, and 3D modeling, and superimpose products on them, providing a realistic virtual representation of how the items would look worn in real life.
Using virtual try-ons makes shopping a breeze and fun, letting folks visualize how the products would look on them without having to try things on in real life. Virtual try-on technologies reduce the number of returns, increase online engagement, and offer a competitive edge in the ecommerce landscape, as well as create unique and compelling reasons for consumers to make in-person visits to stores.
Check out these resources on virtual try-on technologies at popular retailers:
Here are a few papers related to using computer vision for virtual try-ons:
Customer Behavior Analysis
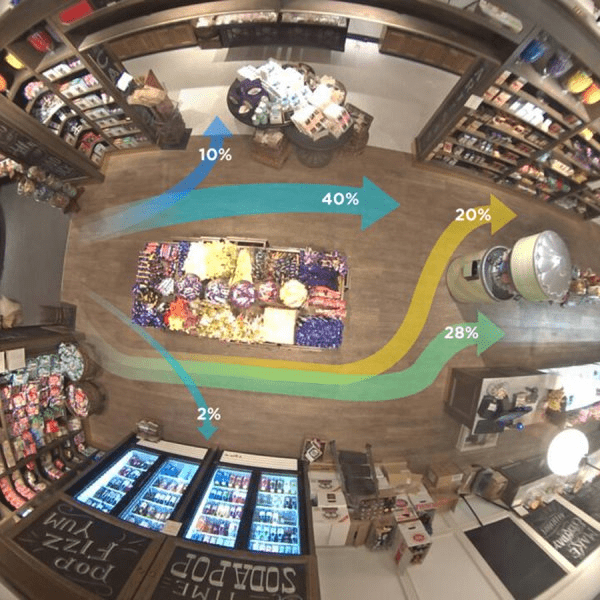
Retail stores can leverage existing CCTV cameras to analyze customer behavior. Based on video footage from these cameras, object tracking algorithms can track customer movements, dwell times, and interactions within the store. This provides insights into shopping patterns, popular areas, and product preferences.
The benefits of understanding customer behavior are substantial. For retailers, it offers actionable insights to optimize store layouts, enhance product placements, and tailor marketing strategies. It also aids in predicting shopping trends, allowing for better inventory management. It results in a more personalized shopping experience, as stores can adjust their offerings and layouts based on observed preferences.
Here are a few papers related to using computer vision for customer behavior analytics:
Product Recommendations
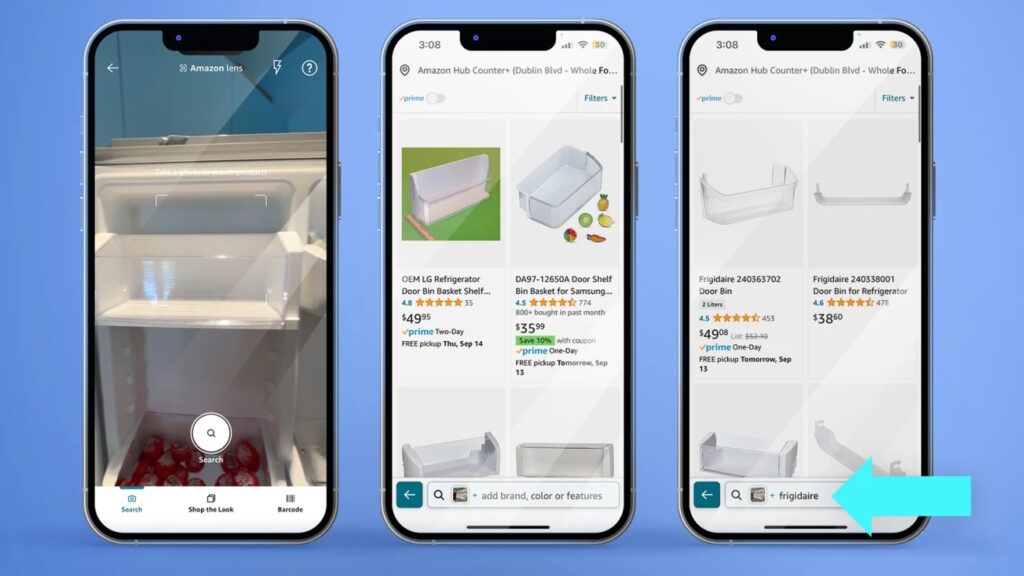
Computer vision enhances product recommendation systems, opening up new possibilities for customer engagement and personalization.
For example, visual search adds a convenient way for consumers to discover new products and information, beyond text searches alone. A growing number of retailers, including IKEA and Amazon through its multimodal (image and text) search, offer the ability for consumers to search for a product by uploading an image.
Recommender systems can present items based on visual similarity to uploaded images, as well additional factors such as recent browsing history, wishlist items, and past purchases, to tailor the shopping experience to an individual consumer’s style and preferences.
Check out these academic papers on computer vision in recommender systems:
Companies at the Cutting Edge of Computer Vision in Retail
Trigo
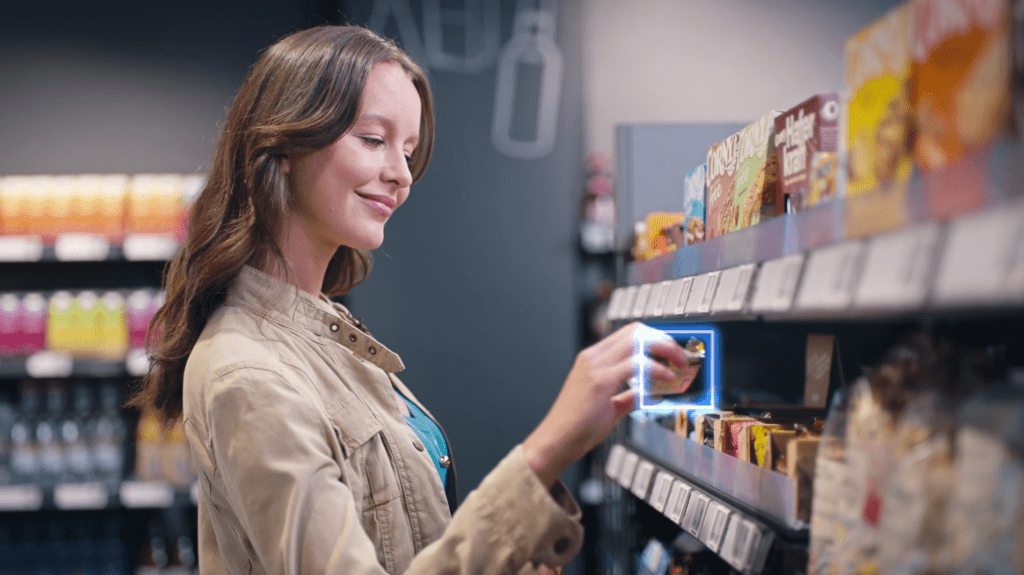
Trigo combines ceiling-based cameras, shelf sensors, and machine vision algorithms to create a digital twin of the retail space. This digital representation allows for real-time analysis of shoppers’ journeys and product choices, enabling better shopping experiences and business outcomes. This setup also enables computer-vision-based autonomous checkout systems that accurately identify and capture the shopping items selected by customers, and make checking out entirely automated. Trigo Vision has attracted significant investments, notably from the German retail giant REWE Group, pushing Trigo’s total fundraising to over $100 million.
Trax Retail
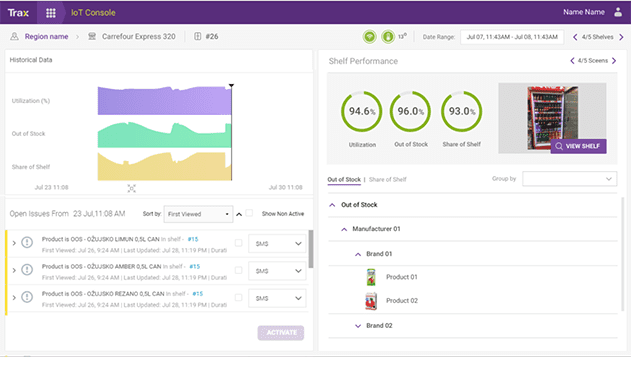
Trax Retail’s mission is to enable brands and retailers to harness the power of digital technologies to produce the best shopping experiences imaginable. Trax’s retail platform allows customers to understand what is happening on-shelf, in every store, all the time so they can focus on what they do best – delighting shoppers. As pioneers in computer vision, Trax continues to lead the industry in innovation and excellence through development of advanced technologies and scalable data collection methods.
Many of the world’s top CPG companies and retailers use Trax’s dynamic merchandising, in-store execution, shopper engagement, market measurement, analytics, and shelf monitoring solutions at scale to drive positive shopper experiences and unlock revenue opportunities at all points of sale.
Link Retail
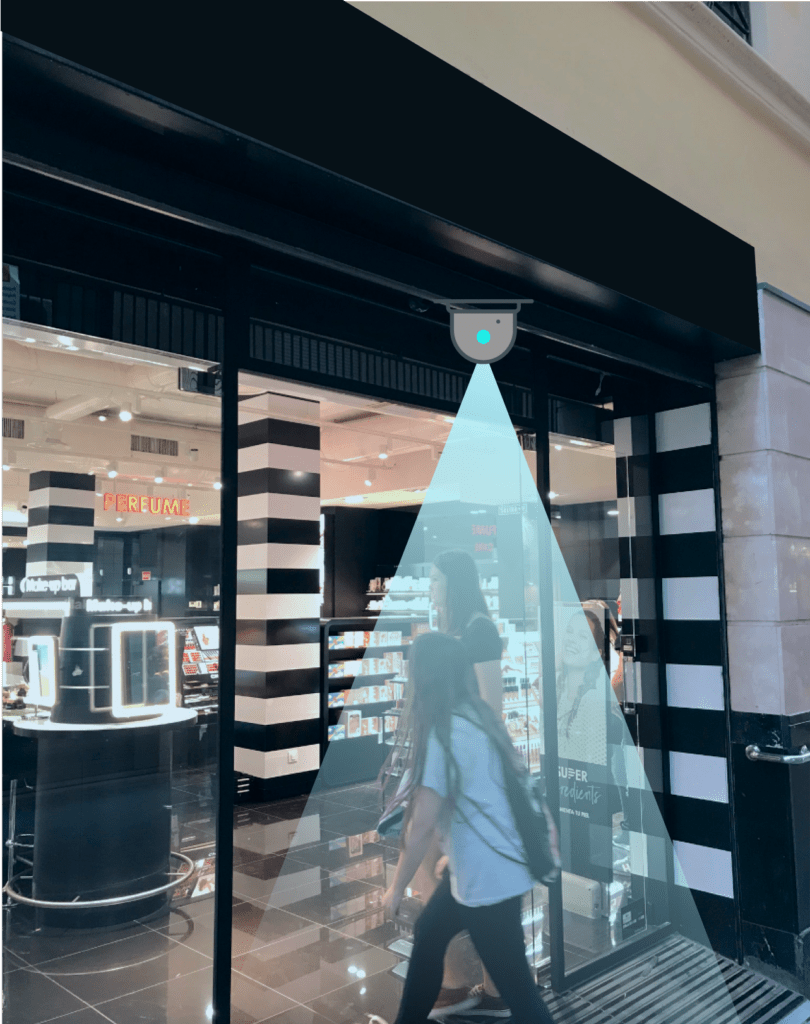
Link Retail, based in Oslo, Norway, uses AI powered techniques to help brick-and-mortar retailers strategically boost sales and optimize operations. The company builds a variety of solutions, including:
-
- Food Waste Management: AI software that optimizes grocery product ordering procedures and reduces retail food waste
-
- Video Analytics: A high accuracy footfall counting system that turns in-store CCTV camera footage into rich operational and shopping data, such as real-time occupancy analysis, shopper flow, and queue analytics
-
- Space Management: An AI analytics tool that employs Point of Sales (POS) data and generates actionable insights on optimizing retail space including floor, shelf, and sales activities
Link Retail helps retailers navigate the challenges of the physical retail environment, making strides toward creating data-driven retail spaces.
Dayta AI
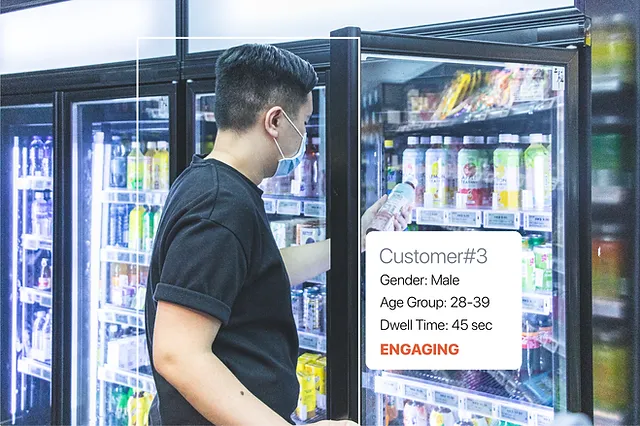
Dayta AI is a retail analytics Software as a Service (SaaS) company that uses computer vision and AI to turn camera footage from retail stores into useful insights. Dayta AI’s solution, Cyclops, is engineered to work with any RTSP-supported cameras, allowing retailers to use their existing video cameras without additional equipment costs. Cyclops can monitor and analyze customer traffic, zone-specific activities, footfall, engagement count, dwell time, queue time, and even emotions, among other metrics. These data points help retailers understand customer behavior, optimize store layouts, and improve the overall customer experience.
RetailNext
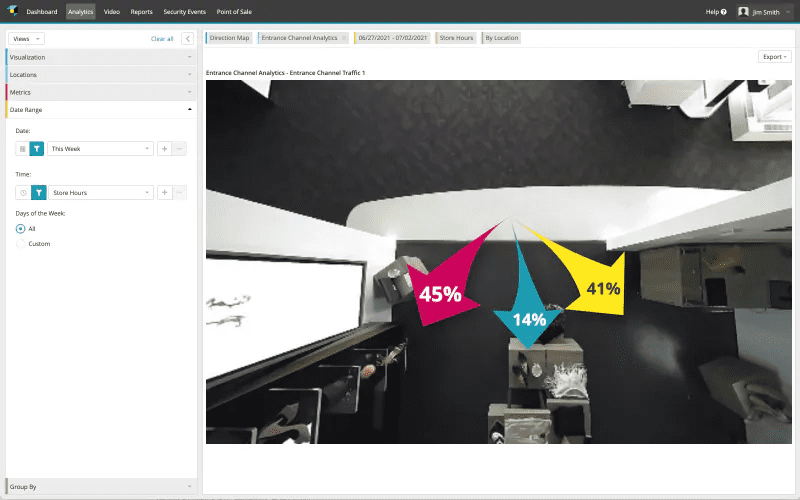
RetailNext was founded to address challenges faced by modern retailers and bring e-commerce style shopper analytics to brick-and-mortar stores, brands, and malls. Through its centralized SaaS platform, RetailNext automatically collects and analyzes shopper behavior data, providing retailers with the critical insights they need to improve the shopper experience in real time. This platform helps retailers optimize store operations, store layouts and marketing strategies, and improve customer experiences. The company also offers a next-generation IoT sensor, Aurora, which is powered by an patented algorithm that uses 3D imagery and deep learning. RetailNext’s technology is trusted by 400+ top retailers and brands globally.
Retail Datasets
If you are interested in exploring applications of computer vision in retail, check out these datasets:
-
- RPC: A Large-Scale and Fine-Grained Retail Product Checkout Dataset: This dataset is designed to advance automatic checkout solutions. It is a collection of 53,739 single-product images in the training set and a combined total of 30,000 multi-product images in the validation and test sets, categorized finely across various product types. Explore the validation set of 6,000 checkout images with FiftyOne in your browser.
-
- Supermarket Shelves Dataset: This dataset can be used to enhance product detection on supermarket shelves. It encompasses 45 images from global supermarkets, amounting to 11,743 bounding boxes, averaging 260 boxes per image.
-
- MERL Shopping: This dataset contains 106 videos of around 2 minutes each from an overhead camera in a grocery setting. It highlights five actions: “Reach To Shelf,” “Retract From Shelf,” “Hand In Shelf,” “Inspect Product,” and “Inspect Shelf.”
-
- Zalando Fashion MNIST: This dataset consists of 70,000 28×28 grayscale images of fashion products categorized into 10 classes like T-shirt/top, trouser, pullover, etc.
-
- GroZi-120: This dataset comprises 120 grocery products captured in both isolated (in vitro) and real-world settings (in situ).
-
- Clothing Coparsing Dataset: This dataset shows the semantic segmentation of different outfits from multiple street fashion models. Explore this dataset with FiftyOne in your browser.
-
- Fashion Product Images: This dataset is a fantastic ecommerce dataset that stores tons of metadata about each article of clothing. The dataset was built for recommender systems that want to take in many different features. Explore this dataset with FiftyOne in your browser.
If you would like to see any of these or other computer vision retail datasets added to the FiftyOne Dataset Zoo, get in touch, and we can work together to make this happen!
Join the FiftyOne Community!
Developers of retail applications can benefit from FiftyOne’s ability to easily filter through the huge amounts of visual data collected daily from stores and other sources. Using open source FiftyOne, this data can be curated into datasets for model training or to share with experts for annotation or analysis of CV models.
Join the thousands of engineers and data scientists already using FiftyOne to solve some of the most challenging problems in computer vision today!
-
- 2,400+ FiftyOne Slack members
-
- 6,350+ stars on GitHub
-
- Used by 470+ repositories
-
- 80+ contributors