Experience ECCV 2024 live, without leaving your desk
Picture this: Milano’s vibrant energy, the world’s top minds in AI/ML gathered in one place, and the future of computer vision unfolding before your eyes. This was ECCV 2024 – and now, we’re bringing highlights from that world-class event to you.
Welcome to the ECCV 2024 Redux series, your virtual pass to some of the groundbreaking research, insights, and innovations that defined this year’s conference. Live streaming from the authors to you.
Customize your registration and attend one, two, or all three days of the series that interest you!
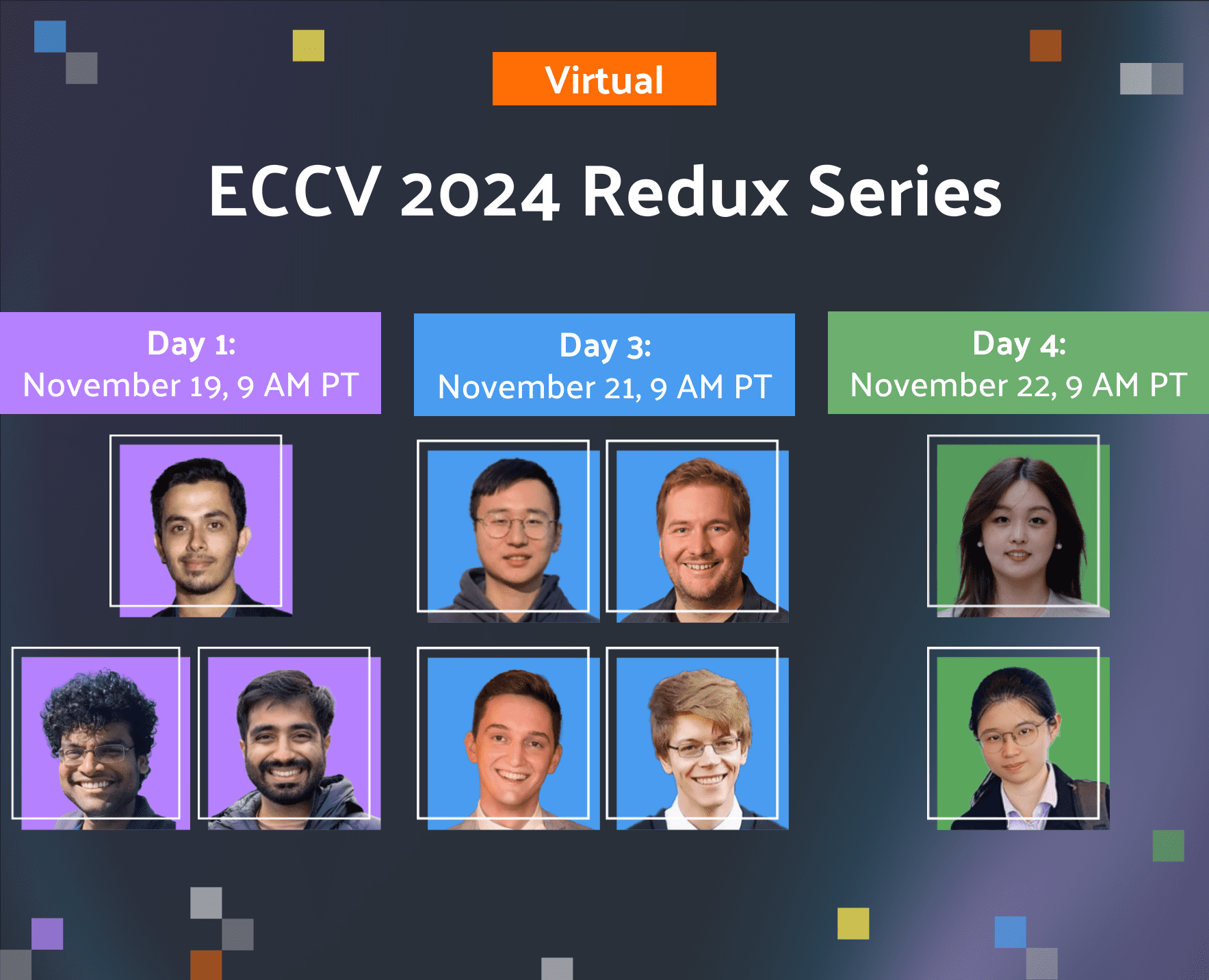
November 19 - “Day 1" Talks & Speakers
Fast and Photo-realistic Novel View Synthesis from Sparse Images
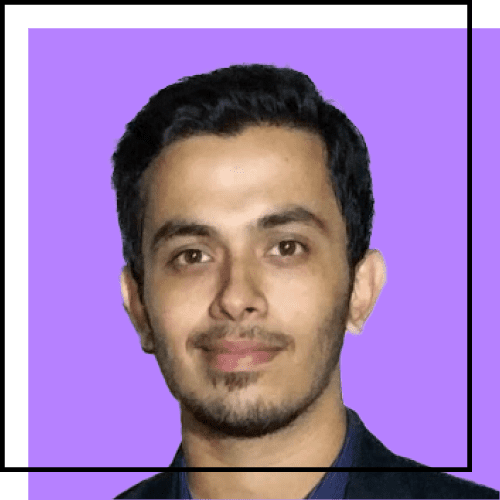
Avinash Paliwal
Texas A&M
Novel view synthesis generates new perspectives of a scene from a set of 2D images, enabling 3D applications like VR/AR, robotics, and autonomous driving. Current state-of-the-art methods produce high-fidelity results but require a lot of images, while sparse-view approaches often suffer from artifacts or slow inference. In this talk, I will present my research work focused on developing fast and photorealistic novel view synthesis techniques capable of handling extremely sparse input views.
ECCV 2024 Paper: CoherentGS: Sparse Novel View Synthesis with Coherent 3D Gaussians
About the Speaker: Avinash Paliwal is a PhD Candidate in the Aggie Graphics Group at Texas A&M University. His research is focused on 3D Computer Vision and Computational Photography.
Robust Calibration of Large Vision-Language Adapters
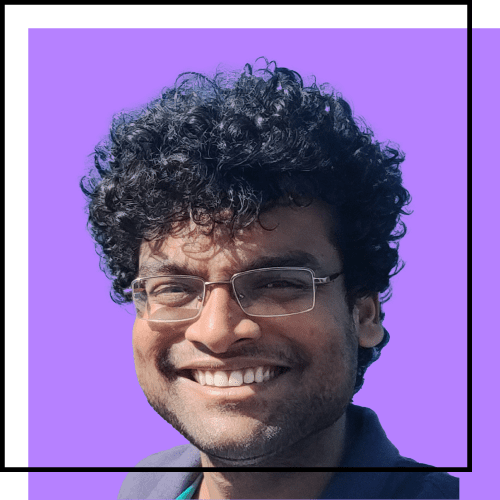
Balamurali Murugesan
ÉTS
We empirically demonstrate that popular CLIP adaptation approaches, such as Adapters, Prompt Learning, and Test-Time Adaptation, substantially degrade the calibration capabilities of the zero-shot baseline in the presence of distributional drift. We identify the increase in logit ranges as the underlying cause of miscalibration of CLIP adaptation methods, contrasting with previous work on calibrating fully-supervised models. Motivated by these observations, we present a simple and model-agnostic solution to mitigate miscalibration, by scaling the logit range of each sample to its zero-shot prediction logits
ECCV 2024 Paper: Robust Calibration of Large Vision-Language Adapters
About the Speaker: Balamurali Murugesan is currently pursuing his Ph.D. in developing reliable deep learning models. Earlier, he completed his master’s thesis on accelerating MRI reconstruction. He has published 25+ research articles in renowned venues.
Tree-of-Life Meets AI: Knowledge-guided Generative Models for Understanding Species Evolution
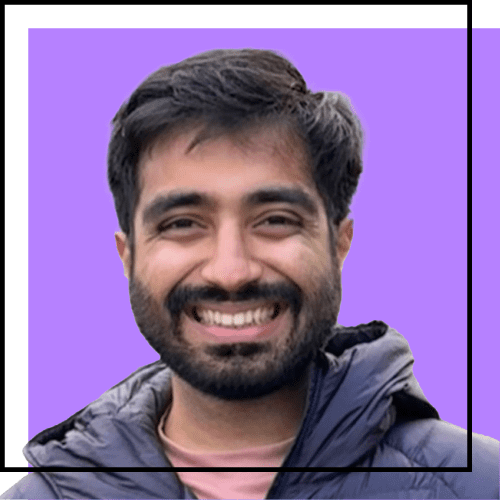
Mridul Khurana
Virginia Tech & NSF
A central challenge in biology is understanding how organisms evolve and adapt to their environment, acquiring variations in observable traits across the tree of life. However, measuring these traits is often subjective and labor-intensive, making trait discovery a highly label-scarce problem. With the advent of large-scale biological image repositories and advances in generative modeling, there is now an opportunity to accelerate the discovery of evolutionary traits. This talk focuses on using generative models to visualize evolutionary changes directly from images without relying on trait labels.
ECCV 2024 Paper: Hierarchical Conditioning of Diffusion Models Using Tree-of-Life for Studying Species Evolution
About the Speaker: Mridul Khurana is a PhD student at Virginia Tech and a researcher with the NSF Imageomics Institute. His research focuses on AI4Science, leveraging multimodal generative modeling to drive discoveries across scientific domains.
November 21 - “Day 3" Talks & Speakers
Closing the Gap Between Satellite and Street-View Imagery Using Generative Models
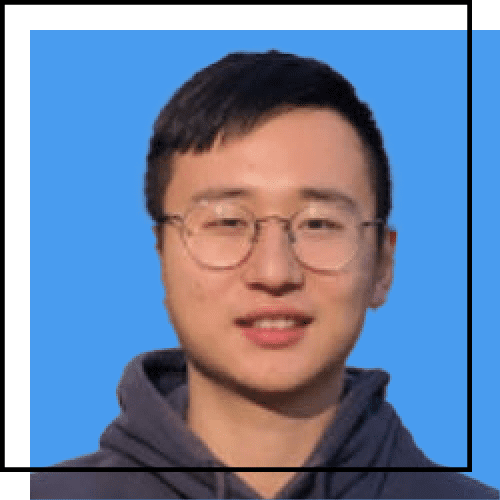
Ningli Xu
Ohio State University
With the growing availability of satellite imagery (e.g., Google Earth), nearly every part of the world can be mapped, though street-view images remain limited. Creating street views from satellite data is crucial for applications like virtual model generation, media content enhancement, 3D gaming, and simulations. This task, known as satellite-to-ground cross-view synthesis, is tackled by our geometry-aware framework, which maintains geometric precision and relative geographical positioning using satellite information.
ECCV 2024 Paper: Geospecific View Generation — Geometry-Context Aware High-resolution Ground View Inference from Satellite Views
About the Speaker: Ningli Xu is a Ph.D. student at The Ohio State University, specializing in generative AI and computer vision, with a focus on addressing image and video generation challenges in the geospatial domain.
High-Efficiency 3D Scene Compression Using Self-Organizing Gaussians
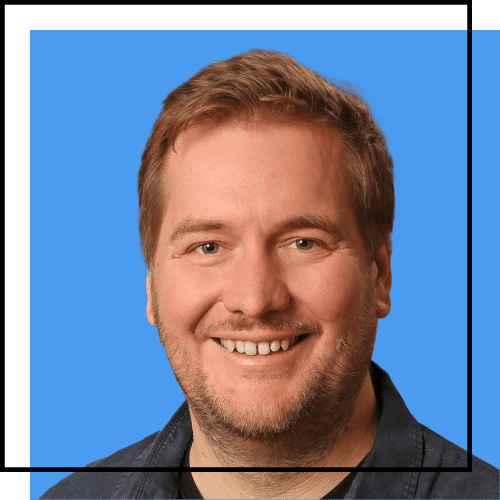
Wieland Morgenstern
Fraunhofer Heinrich Hertz Institute
In just over a year, 3D Gaussian Splatting (3DGS) has made waves in computer vision for its remarkable speed, simplicity, and visual quality. Yet, even scenes of a single room can exceed a gigabyte in size, making it difficult to scale up to larger environments, like city blocks. In this talk, we’ll explore compression techniques to reduce the 3DGS memory footprint. We’ll dive deeply into our novel approach, Self-Organizing Gaussians, which proposes to map splatting attributes into a 2D grid, using a high-performance parallel linear assignment sorting developed to reorganize the splats on the fly. This grid assignment allows us to leverage traditional 2D image compression techniques like JPEG to efficiently store 3D data. Our method is quick and easy to decompress and provides a surprisingly competitive compression ratio. The drastically reduced memory requirements make this method perfect for efficiently streaming 3D scenes at large scales, which is especially useful for AR, VR and gaming applications.
ECCV 2024 Paper: Compact 3D Scene Representation via Self-Organizing Gaussian Grids
About the Speaker: Wieland Morgenstern is a Research Associate at the Computer Vision & Graphics group at Fraunhofer HHI and is pursuing a PhD at Humboldt University Berlin. His research focuses on representing 3D scenes and virtual humans.
Skeleton Recall Loss for Connectivity Conserving and Resource Efficient Segmentation of Thin Tubular Structures
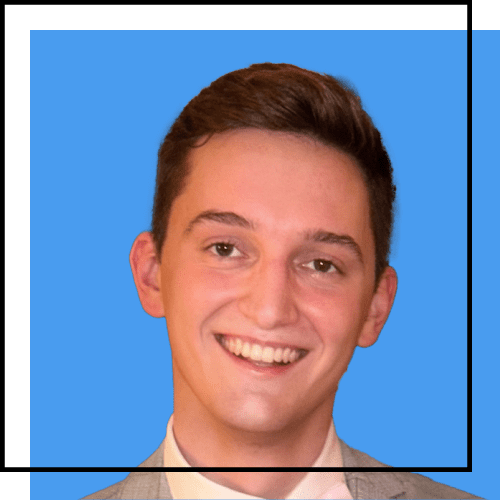
Maximilian Rokuss
Medical Image Computing at German Cancer Research Center & Heidelberg University
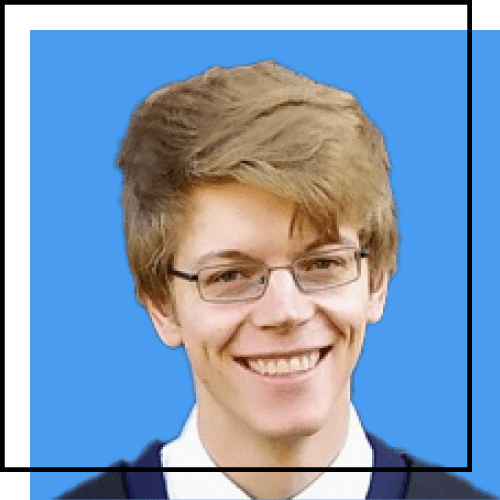
Yannick Kirchoff
Medical Image Computing at German Cancer Research Center & Heidelberg University
We present Skeleton Recall Loss, a novel loss function for topologically accurate and efficient segmentation of thin, tubular structures, such as roads, nerves, or vessels. By circumventing expensive GPU-based operations, we reduce computational overheads by up to 90% compared to the current state-of-the-art, while achieving overall superior performance in segmentation accuracy and connectivity preservation. Additionally, it is the first multi-class capable loss function for thin structure segmentation.
ECCV 2024 Paper: Skeleton Recall Loss for Connectivity Conserving and Resource Efficient Segmentation of Thin Tubular Structures
About the Speakers:
Maximilian Rokuss holds a M.Sc. in Physics from Heidelberg University, now PhD Student in Medical Image Computing at German Cancer Research Center (DKFZ) and Heidelberg University
Yannick Kirchoff holds a M.Sc. in Physics from Heidelberg University, now PhD Student in Medical Image Computing at German Cancer Research Center (DKFZ) and Helmholtz Information and Data Science School for Health
November 22 - “Day 4" Talks & Speakers
Zero-shot Video Anomaly Detection: Leveraging Large Language Models for Rule-Based Reasoning
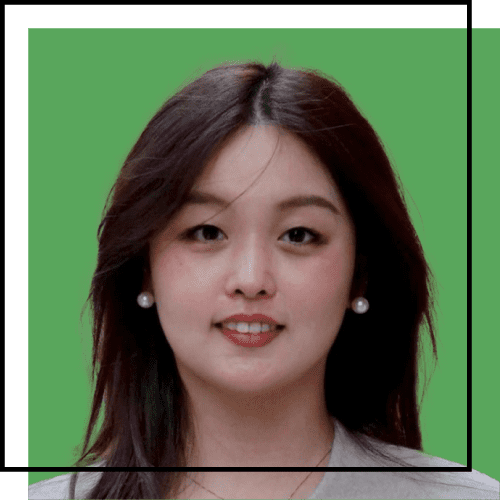
Yuchen Yang
John Hopkins University
Video Anomaly Detection (VAD) is critical for applications such as surveillance and autonomous driving. However, existing methods lack transparent reasoning, limiting public trust in real-world deployments. We introduce a rule-based reasoning framework that leverages Large Language Models (LLMs) to induce detection rules from few-shot normal samples and apply them to identify anomalies, incorporating strategies such as rule aggregation and perception smoothing to enhance robustness. The abstract nature of language enables rapid adaptation to diverse VAD scenarios, ensuring flexibility and broad applicability.
ECCV 2024 Paper: Follow the Rules: Reasoning for Video Anomaly Detection with Large Language Models
About the Speaker: Yuchen Yang is a a Ph.D. Candidate in the Department of Computer Science at Johns Hopkins University. Her research aims to deliver functional, trustworthy solutions for machine learning and AI systems.
Open-Vocabulary 3D Semantic Segmentation with Text-to-Image Diffusion Models
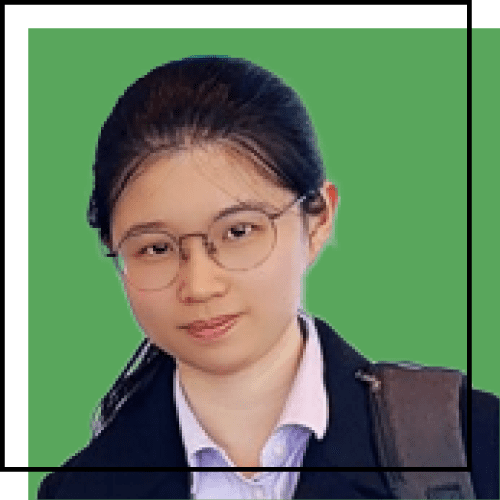
Xiaoyu Zhu
Carnegie Mellon
In this talk, I will introduce our recent work on open-vocabulary 3D semantic understanding. We propose a novel method, namely Diff2Scene, which leverages frozen representations from text-image generative models, for open-vocabulary 3D semantic segmentation and visual grounding tasks. Diff2Scene gets rid of any labeled 3D data and effectively identifies objects, appearances, locations and their compositions in 3D scenes.
ECCV 2024 Paper: Open-Vocabulary 3D Semantic Segmentation with Text-to-Image Diffusion Models
About the Speaker: Yuchen Yang is a a Ph.D. Candidate in the Department of Computer Science at Johns Hopkins University. Her research aims to deliver functional, trustworthy solutions for machine learning and AI systems.