Navigating the complexities of medical image analysis has always been a formidable challenge, especially in the face of domain shifts.
As deep learning revolutionizes image recognition across various fields, its application in healthcare remains an intricate task due to these shifts. In this post, we chat with the author of a breakthrough paper, “A Textbook Remedy for Domain Shifts: Knowledge Priors for Medical Image Analysis”. This research offers a ground-breaking solution, Knowledge Enhanced Bottlenecks (KnoBo), which promises to bolster the robustness of models used in medical image analysis.
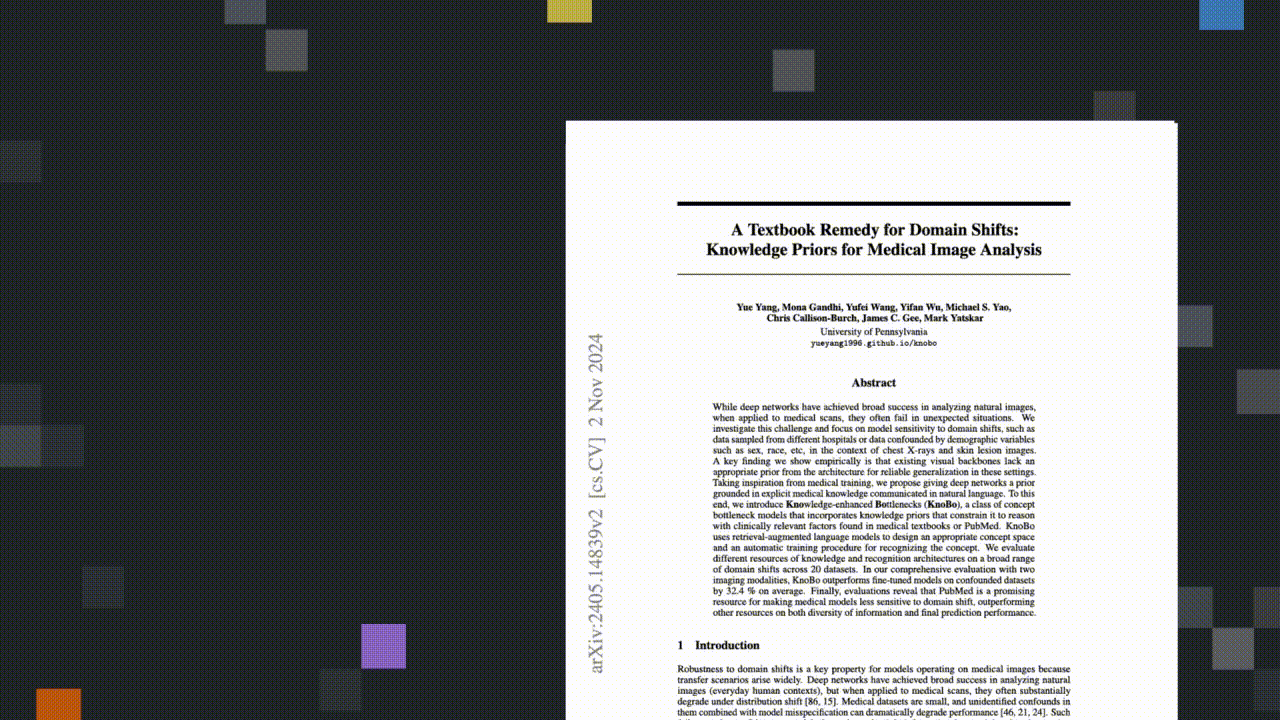
NeurIPS 2024 Paper: A Textbook Remedy for Domain Shifts: Knowledge Priors for Medical Image Analysis
Author: Yue Yang, PhD student in NLP/CV at University of Pennsylvania.
Understanding Domain Shifts
Domain shifts arise from discrepancies between training data and real-world data encountered during deployment.
One reason for the vulnerability of deep learning models to domain shifts is the lack of effective deep image priors for medical images. Deep image priors refer to data-agnostic assumptions about the task embedded in a model’s architecture. They allow models to generalize to new data even without prior exposure.
This inconsistency threatens the accuracy of diagnoses and, consequently, patient care.
Challenges in Medical Image Analysis
Medical image data presents unique challenges, including privacy concerns and demographic biases, which complicate scaling and effective model deployment.
Currently, many deep learning architectures, designed for general domains, are ill-suited for the medical field. Existing models often exploit confounding factors like sex, age, or race, leading to significant performance drops in unexpected data configurations.
This lack of robustness is a serious problem for patient care, as inaccurate diagnoses can lead to negative outcomes.
Deep Image Priors: A Double-Edged Sword
Traditional models like CNNs or Vision Transformers benefit from deep image priors, which are the inherent assumptions that aid natural image processing.
However, these priors are less effective for medical images, which require more nuanced analysis beyond what general architectures can provide. This suggests that the architectural assumptions baked into these models are not well-suited for interpreting medical images, leading to over-reliance on training data and a susceptibility to spurious correlations.
KnoBo addresses this gap by aligning model features with medically relevant concepts extracted from foundational knowledge resources.
Building a Robust Medical AI Model
Yue Yang’s research draws inspiration from the educational journey of medical professionals, who master foundational knowledge from textbooks before applying it in real-world scenarios. KnoBo models enhance robustness by integrating this structured approach, outperforming traditional deep learning models across diverse medical image datasets. This innovation improves model accuracy in varying data environments, and builds trust and adoption of AI in healthcare by delivering interpretable results.
How Does KnoBo Work?
KnoBo incorporates explicit medical knowledge into the model, mimicking the way medical professionals learn.
Rather than relying solely on visual data, KnoBo incorporates knowledge from medical textbooks and articles, using this foundational knowledge to interpret patient cases. KnoBo’s approach involves constructing concept bottlenecks by harnessing medical texts, such as PubMed articles, to extract relevant diagnostic knowledge. This structured knowledge informs model architecture, creating a robust system grounded in medical expertise rather than solely data patterns.
Here’s a step-by-step breakdown of the KnoBo system:
- Structure Prior: A retrieval-augmented generation system extracts relevant concepts from medical documents like PubMed articles, textbooks, and Wikipedia entries. These concepts are formulated as binary questions (e.g., “Is there ground-glass opacity?”) and serve as a knowledge base for the model.
- Bottleneck Predictor: Each concept is then “grounded” to the images by training a binary classifier that predicts the presence or absence of the concept in an image. This training leverages a pre-existing dataset of medical images and their associated clinical reports.
- Parameter Prior: Finally, a linear layer combines the concept predictions to make the final label prediction. This layer is constrained by a parameter prior that encodes known relationships between concepts and labels. For example, the model might be informed that “ground-glass opacity” is positively correlated with a diagnosis of COVID-19
KnoBo outperforms conventional deep learning approaches on various medical image datasets, particularly in situations with domain shifts. Beyond it’s improved accuracy, the model’s reliance on explicit medical concepts makes its decision-making process more transparent and understandable to clinicians, potentially increasing trust and facilitating broader adoption of AI in healthcare.
Expanding KnoBo’s Horizons
Despite its promise, KnoBo is not without limitations.
- Dependence on Labeled Medical Datasets: KnoBo still requires large, labeled medical datasets for concept grounding, which can be scarce for rare conditions.
- Limited Feature Extractor Capabilities: The lack of powerful medical image feature extractors currently limits the performance of concept predictors in KnoBo. Future research in medical foundation models could provide better feature representations and further enhance KnoBo’s performance.
There’s potential for integrating diverse data sources, such as wearable tech or genomic data, into the KnoBo framework. This multimodal approach aligns with how doctors synthesize information from multiple tests and observations to form a diagnosis.
By leveraging broader datasets and building superior feature representations, KnoBo could potentially close the performance gap further and enhance interpretability
Application in Medical Education
KnoBo’s interpretability offers an interactive tool for medical education, allowing students to learn from AI just as junior doctors learn from seniors. Future developments could use KnoBo to provide personalized feedback and aid in understanding complex medical concepts, transforming the educational landscape in medicine.
Conclusion
KnoBo stands at the forefront of enhancing AI’s role in healthcare, offering robust models that adapt gracefully across diverse data conditions. As healthcare continues embracing AI, solutions like KnoBo promise improved outcomes and broader confidence among clinicians and technologists. Meeting Yue Yang and his team at NeurIPS will be a significant step toward integrating their findings into the broader AI healthcare community.
We look forward to further developments in this exciting field and invite everyone to explore these findings in greater depth at the NeurIPS conference, where innovation is poised to redefine the future of medical image analysis.