Boston AI, ML and Computer Vision Meetup
Feb 28, 2025 | 5:00 to 8:00 PM
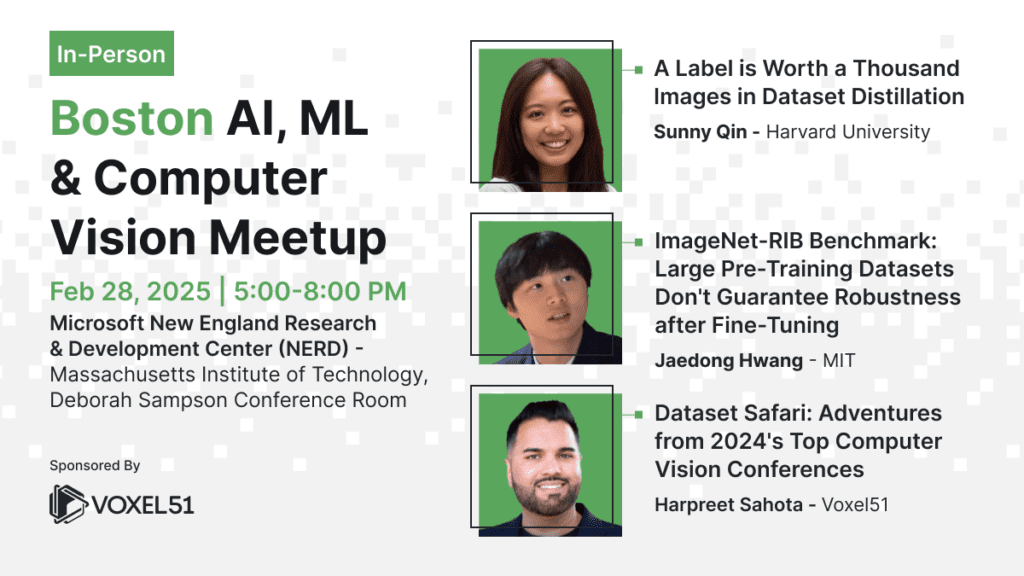
Register for the event at Microsoft NERD
By submitting you (1) agree to Voxel51’s Terms of Service and Privacy Statement and (2) agree to receive occasional emails.
Date, Time and Location
Date and Time
Feb 28, 2025 from 5:00 to 8:00 PM
Location
The Meetup will take place at Microsoft Research Lab – New England (NERD) at MIT, One Memorial Drive, Cambridge, MA, 02142.
A Label is Worth a Thousand Images in Dataset Distillation
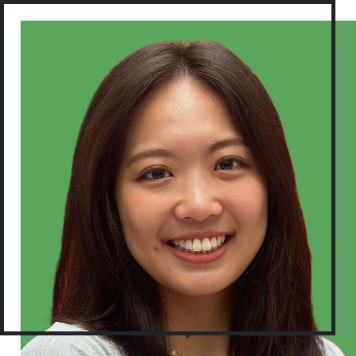
Sunny Qin
Harvard University
Dataset distillation is a method to create smaller yet highly informative datasets that retain the efficacy of larger datasets. In this talk, I’ll highlight the importance of soft labels—probabilistic labels that provide more nuanced information than hard labels—in improving model performance. We’ll also cover the relationship between data and knowledge distillation, the role of expert-generated labels, and the challenges and benefits of using soft labels, even in noisy data scenarios.
About the Speaker
Sunny Qin a 3rd year graduate student at Harvard. I am advised by David Alvarez-Melis and am part of the Machine Learning Foundations Group. I am interested in data-centric machine learning, mechanistic interpretability, and their intersections.
ImageNet-RIB Benchmark: Large Pre-Training Datasets Don't Guarantee Robustness after Fine-Tuning
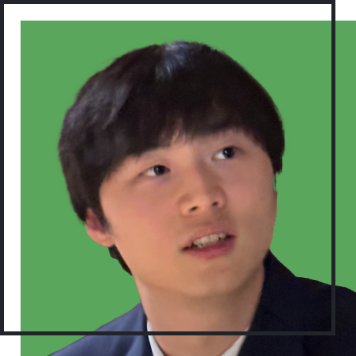
Jaedong Hwang
MIT
Highly performant large-scale pre-trained models promise to provide a valuable foundation for learning specialized tasks through fine-tuning. By starting with a robust general-purpose model, the goal is to achieve both specialization in the target task and to maintain robustness. To evaluate model robustness to out-of-distribution samples after fine-tuning on downstream datasets, we introduce a new benchmark, ImageNet-RIB (Robustness Inheritance Benchmark). This benchmark comprises a set of related but distinct specialized tasks; pre-trained models are fine-tuned on one task in the set, and their robustness is evaluated on the others, iterating across all tasks. Counterintuitively, we find that model robustness after fine-tuning on related downstream tasks is lowest when the pre-training dataset is the richest and most diverse. This result suggests that starting with the strongest foundation model may not always be the best strategy for achieving optimal performance on specialist tasks.
About the Speaker
Jaedong Hwang is a Ph.D. student in the Department of Electrical Engineering and Computer Science (EECS) at the Massachusetts Institute of Technology (MIT), advised by Professor Ila Fiete. His research focuses on learning from imperfect supervision, building efficient AI inspired by neuroscience, and advancing neuroscience research through AI. He has received multiple Outstanding Reviewer Awards from CVPR, ICCV, and ICLR. Previously, he interned at Adobe Research and earned his M.S. in Electrical and Computer Engineering and B.S. in Computer Science and Engineering from Seoul National University, specializing in computer vision.
Dataset Safari: Adventures from 2024's Top Computer Vision Conferences
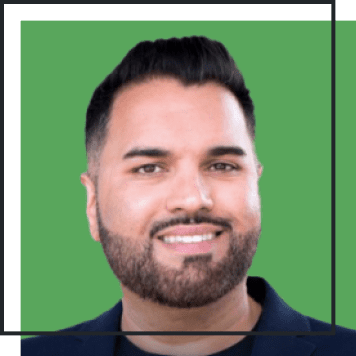
Harpreet Sahota
Voxel51
Datasets are the lifeblood of machine learning, driving innovation and enabling breakthrough applications in computer vision and AI. This talk presents a curated exploration of the most compelling visual datasets unveiled at CVPR, ECCV, and NeurIPS 2024, with a unique twist – we’ll explore them live using FiftyOne, the open-source tool for dataset curation and analysis.
Using FiftyOne’s powerful visualization and analysis capabilities, we’ll take a deep dive into these collections, examining their unique characteristics through interactive sessions. We’ll demonstrate how to:
- Analyze dataset distributions and potential biases
- Identify edge cases and interesting samples
- Compare similar samples across datasets
- Explore multi-modal annotations and complex label structures
Whether you’re a researcher, practitioner, or dataset enthusiast, this session will provide hands-on insights into both the datasets shaping our field and practical tools for dataset exploration. Join us for a live demonstration of how modern dataset analysis tools can unlock deeper understanding of the data driving AI forward.
About the Speaker
Harpreet Sahota is a hacker-in-residence and machine learning engineer with a passion for deep learning and generative AI. He’s got a deep interest in RAG, Agents, and Multimodal AI.
Find a Meetup Near You
Join the AI and ML enthusiasts who have already become members
The goal of the AI, Machine Learning, and Computer Vision Meetup network is to bring together a community of data scientists, machine learning engineers, and open source enthusiasts who want to share and expand their knowledge of AI and complementary technologies. If that’s you, we invite you to join the Meetup closest to your timezone.