Ann Arbor AI, Machine Learning and Computer Vision Meetup
Jan 16, 2025 | 5:30 to 8:30 PM
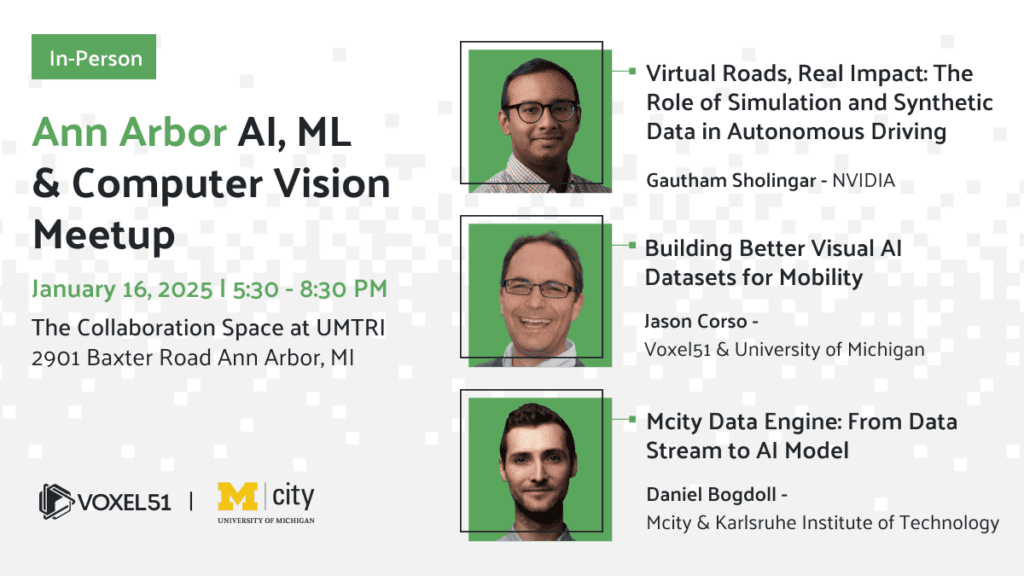
Register for the event at Fora Space
By submitting you (1) agree to Voxel51’s Terms of Service and Privacy Statement and (2) agree to receive occasional emails.
Date, Time and Location
Date and Time
Jan 16, 2025 from 5:30 PM to 8:30 PM
Location
The Collaboration Space at UMTRI, 2901 Baxter Rd, Ann Arbor, MI
Virtual Roads, Real Impact: The Role of Simulation and Synthetic Data in Autonomous Driving
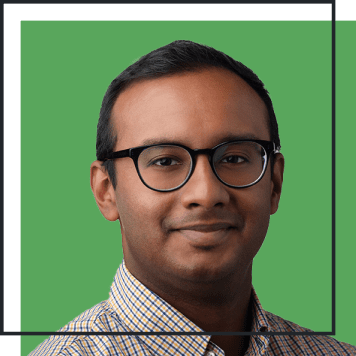
Gautham Sholingar
NVIDIA
As the generative AI revolution unfolds, autonomous vehicles are experiencing a paradigm shift from rule-based stacks to end-to-end architectures. This transition creates a need for massive amounts of sensor data for model training and validation. Sensor simulation becomes essential to augment real world datasets to meet these complex and diverse data needs. Learn how NVIDIA is bringing together physically accurate sensor simulation with existing developer workflows and collaborating with ecosystem partners to accelerate autonomous vehicle deployment.
About the Speaker
Gautham Sholingar is a Senior Manager leading AV Simulation product management for NVIDIA Omniverse. Gautham has several years of experience working in simulation, AI/machine learning, and automated driving, with prior roles at Ford Motor Company and MathWorks. Gautham holds a bachelor’s degree in electrical engineering from the University of Michigan, a master’s degree in electrical engineering from the California Institute of Technology, and an MBA from the Haas School of Business, University of California, Berkeley.
Mcity Data Engine: From Data Stream to AI Model
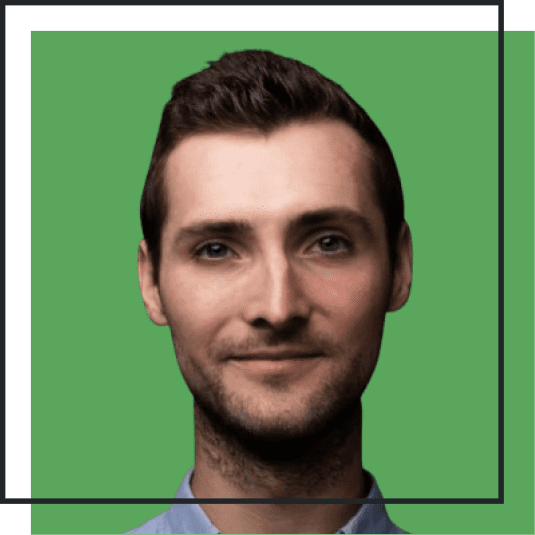
Daniel Bogdoll
Mcity and Karlsruhe Institute of Technology
The Mcity data engine is designed to train AI models on large volumes of raw sensor data. For our current use case, data is collected from roadside fisheye cameras that capture traffic at intersections. FiftyOne is used to curate the data and select diverse samples for labeling. The labeled dataset is then used to train object detectors/trackers/predictors of vehicles, bicyclists, and pedestrians.
About the Speaker
Daniel Bogdoll is a visiting research scholar at Mcity and PhD student at Karlsruhe Institute of Technology, Germany. He has been working in the field of mobility for 9 years and currently focuses on detecting unusual scenarios.
Building Better Visual AI Datasets for Mobility
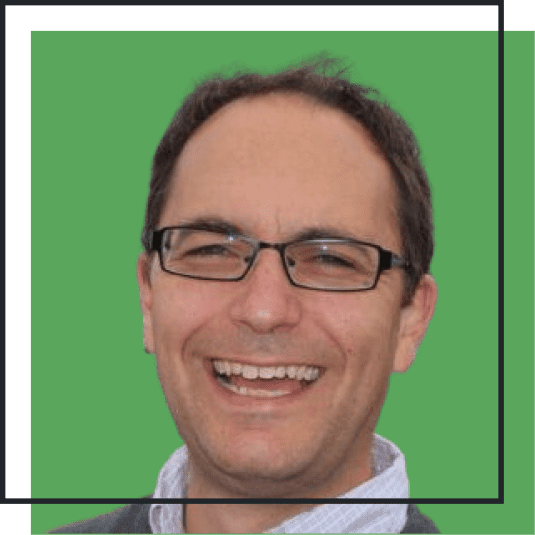
Prof Jason Corso
Voxel51 and University of Michigan
Building datasets for training visual AI models is laborious, error-prone, and expensive. Even worse, we lack the ability to measure how good a dataset is for a certain task. And, yet, we spend immense amounts of capital on building datasets, especially the case in the automotive sector. This talk will explore two vectors of inquiry around improving our ability to build measurably high-quality datasets for training models. First, I will explore discuss new methods in pruning large data-lakes down to size for model training guided by the ability to estimate dataset difficulty. Then, I will describe the potential for AI-human teaming when building and annotating datasets, leveraging a human in the loop to guide auto-labeling models when labeling complex scene geometry for mobility datasets.
About the Speaker
Dr. Jason Corso is a professor of electrical engineering and computer science at the University of Michigan and co-founder and CEO of Voxel51, an AI software company creating dev tools for improving the performance of computer vision and machine learning systems.
Find a Meetup Near You
Join the AI and ML enthusiasts who have already become members
The goal of the AI, Machine Learning, and Computer Vision Meetup network is to bring together a community of data scientists, machine learning engineers, and open source enthusiasts who want to share and expand their knowledge of AI and complementary technologies. If that’s you, we invite you to join the Meetup closest to your timezone.